AI predicts patient’s race from x-ray images with 99% accuracy – is that as good thing?
New research has found that AI analyzing medical scans can identify the race of patients with an astonishing degree of accuracy.
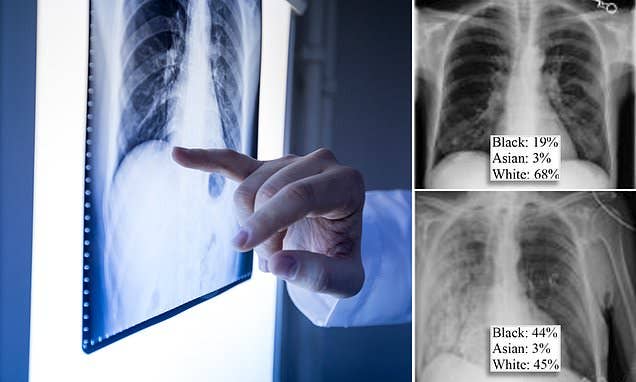
[August 26, 2021: James Felton]
New research has found that artificial intelligence (AI) analyzing medical scans can identify the race of patients with an astonishing degree of accuracy, while their human counterparts cannot. With the Food and Drug Administration (FDA) approving more algorithms for medical use, the researchers are concerned that AI could end up perpetuating racial biases. They are especially concerned that they could not figure out precisely how the machine-learning models were able to identify race, even from heavily corrupted and low-resolution images.
In the study, published on pre-print service Arxiv, an international team of doctors investigated how deep learning models can detect race from medical images. Using private and public chest scans and self-reported data on race and ethnicity, they first assessed how accurate the algorithms were, before investigating the mechanism.
"We hypothesized that if the model was able to identify a patient's race, this would suggest the models had implicitly learned to recognize racial information despite not being directly trained for that task," the team wrote in their research.
They found, as previous studies had, that the machine-learning algorithms were able to predict with high accuracy whether the patients were Black, White, or Asian. The team then tested a number of possible ways that the algorithm could glean this information.
Among the proposed ideas was that the AI could pick up differences in the density of breast tissue or bone. However, when these factors were masked (by clipping pixel brightness at 60 percent for bone density), the AI was still able to predict with accuracy the self-reported race of the patients.
Other possibilities included the AI guessing from regional differences in markers on the scan (say one hospital that sees a lot of white patients marks their X-Rays in a specific style, it may be able to guess from demographics), or that there were differences in how high-resolution the scans were when they were taken (for example, deprived areas may not have as good equipment). Again, these factors were controlled for through heavily pixelating, cropping, and blurring the images. The AI could still predict ethnicity and race when humans could not.
Even when the resolution of the scan was reduced to 4 x 4 pixels, the predictions were still better than random chance – and by the time resolution was increased to 160 x 160 pixels, accuracy was over 95 percent.
"Models trained on high-pass filtered images maintained performance well beyond the point that the degraded images contained no recognisable structures," they write. "To the human co-authors and radiologists it was not even clear that the image was an x-ray at all."
Other variables were tested, and the results came back the same.
"Overall, we were unable to isolate image features that are responsible for the recognition of racial identity in medical images, either by spatial location, in the frequency domain, or caused by common anatomic and phenotype confounders associated with racial identity."
AI can guess your ethnicity, and the people who trained it don't know how. The team is concerned that the inability to anonymize this information from AI could lead to further disparities in treatment.
"These findings suggest that not only is racial identity trivially learned by AI models, but that it appears likely that it will be remarkably difficult to debias these systems," they explain. "We could only reduce the ability of the models to detect race with extreme degradation of the image quality, to the level where we would expect task performance to also be severely impaired and often well beyond that point that the images are undiagnosable for a human radiologist."
Machine learning algorithms have already been shown to be fallible in this area. In 2019 an algorithm widely used to prioritise care for seriously ill patients was shown to disadvantaged Black patients, while in 2020 one algorithm consistently assigned lower risk scores to Black patients with kidney disease, downplaying the seriousness of their disease. Another, trained to flag pneumonia and other chest conditions, performed differently for people of different sexes, ages, races, and types of medical insurance.
The authors note that thus far, regulators haven't taken into account unexpected racial biases within AI, nor produced processes that can guard against harms that are produced by biases within models.
"We strongly recommend that all developers, regulators, and users who are involved with medical image analysis consider the use of deep learning models with extreme caution," the authors conclude. "In the setting of x-ray and CT imaging data, patient racial identity is readily learnable from the image data alone, generalises to new settings, and may provide a direct mechanism to perpetuate or even worsen the racial disparities that exist in current medical practice."
Like these kind of feel good stories? Get the Brighter Side of News' newsletter.
Tags: #New_Innovations, #XRays, #AI, #Medical_News, #The_Brighter_Side_of_News
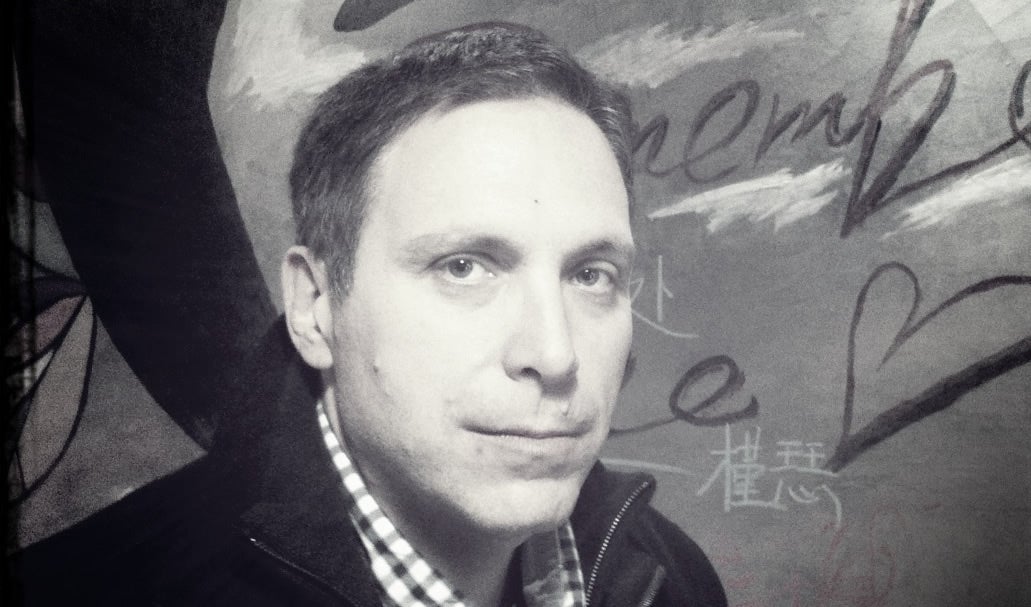