Artificial intelligence can now create AI applications on its own
A prominent player in artificial intelligence (AI) for edge applications, has unveiled a paradigm-shifting development in the AI field
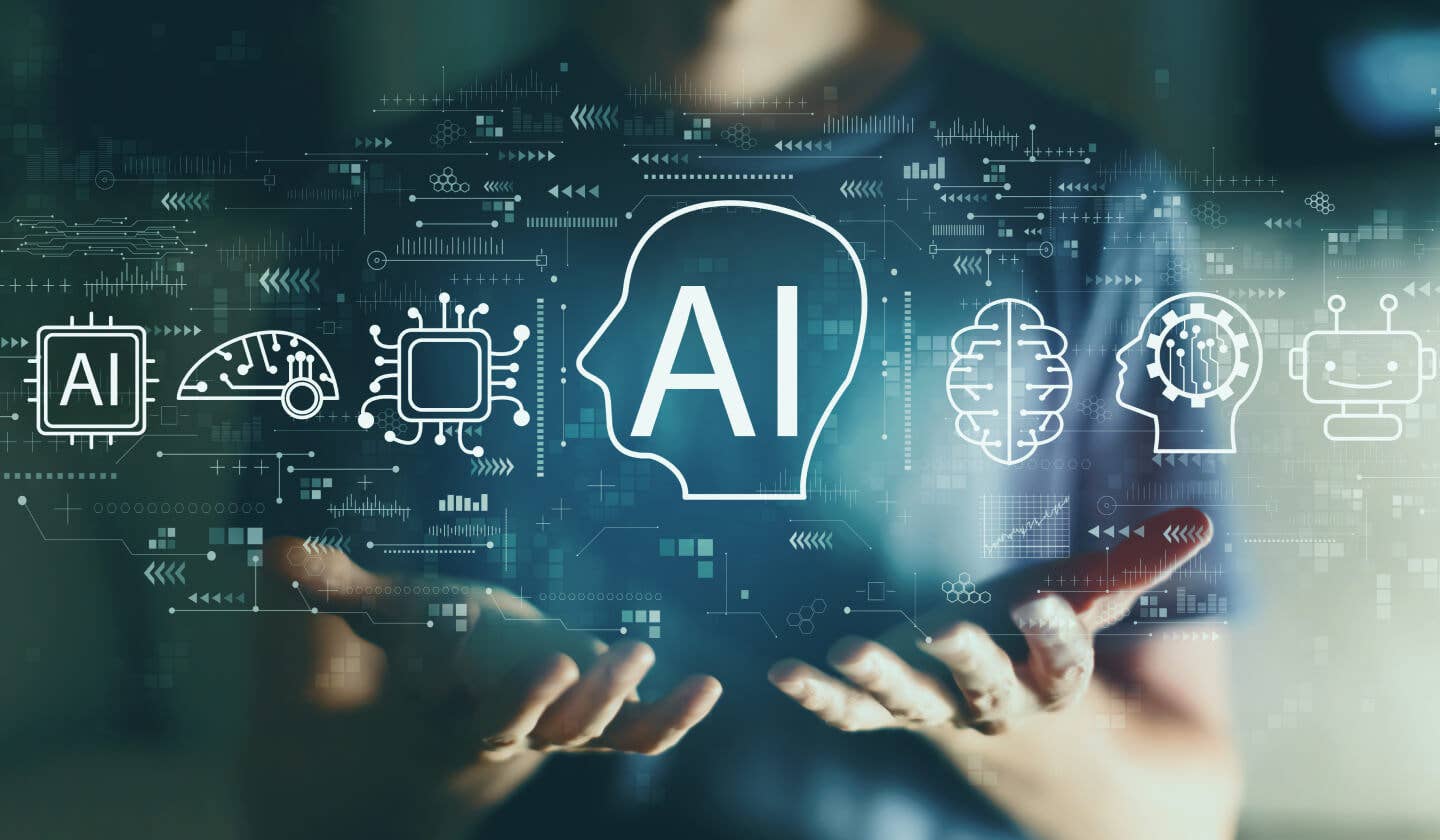
Alzip Inc. has unveiled a paradigm-shifting development in the AI field: the ability to create AI models using AI models. (CREDIT: Creative Commons)
In a groundbreaking announcement, Aizip, Inc., a prominent player in artificial intelligence (AI) for edge applications, has unveiled a paradigm-shifting development in the AI field: the ability to create AI models using AI models.
This groundbreaking achievement is the result of a collaborative effort between Aizip and a team of esteemed scientists from MIT, UC Berkeley, UC Davis, and UC San Diego. Together, they have introduced Aizipline, a fully automated AI-design pipeline that spans data generation, model design, and model testing, ushering in a new era of AI-powered innovation.
The advent of foundation models and generative AI, exemplified by models like ChatGPT, has garnered global attention and opened doors to an array of exciting applications. From content generation to AI-driven coding, these technologies have demonstrated remarkable progress, pushing the boundaries of what AI can accomplish. However, the concept of AI developing other AI models, long relegated to the realm of science fiction, has become a reality through Aizip's pioneering efforts.
The interdisciplinary team behind Aizip's AI-design automation paradigm has harnessed the power of AI to create efficient models automatically, employing a spectrum of cutting-edge techniques.
Related Stories:
These techniques include unsupervised representation learning, generative models, transfer learning, efficient neural architectures, neural architecture search, hardware-aware training, and sim2real testing. By integrating data-centric, model-centric, and system-centric approaches, the team has achieved unparalleled efficiency across a wide range of AI applications.
One standout example of this technical prowess is the fully automated keyword spotting (KWS) design pipeline. By leveraging generative models and scalable neural architecture search, Aizip has streamlined the development of highly efficient KWS models that excel under diverse acoustic conditions.
The resulting AI-generated knowledge can be encapsulated into economical models, delivering maximal efficiency. The ultimate vision of this AI-design automation paradigm is the creation of an "AI Nanofactory," capable of generating millions of specialized, efficient AI models with minimal human intervention, poised to drive the future of pervasive AI and AI engineering.
Aizip's initial foray into the burgeoning pervasive intelligence market underscores the potential for small models to outpace larger ones, thanks to the assistance of foundation models. Brian Cheung, an AI scientist at MIT and Chief Scientist of Aizip, explains, "With the help of large foundation models, small models will evolve faster than big ones, so the trend of improvements favors the edge.
This is a space where Aizip has been an early adopter, and Aizip now leads in leveraging the big to dramatically improve the small." Aizip's intensive work over the past three years has yielded a suite of tools harnessing generative AI, foundation models, vector databases, self-supervised learning, and efficient neural architectures, among other state-of-the-art AI advancements. These tools have the potential to significantly shorten or even fully automate the AI-model development cycle.
Boltzmann Li, Principal Machine Learning Architect at Aizip, provides an example of Aizip's approach: "Let's take keyword spotting as an example. To develop a customized and ultra-efficient KWS model that can perform reliably under a variety of conditions, Aizip leveraged generative models to synthesize keywords with strong diversity in speaker identity, acoustic environments, noise conditions, and other factors."
Combining generative models with ultra-efficient neural architectures, Aizip has achieved full automation in the KWS model development process. While some applications still require human involvement, Aizip's ultimate goal remains complete AI design automation (ADA).
The inspiration for Aizip's approach comes from nature itself. Bruno Olshausen, a professor at UC Berkeley and Director of the Redwood Center for Theoretical Neuroscience, draws parallels between efficient AI systems and the neural networks of small animals.
"Just as the tiny brains of small animals with fewer than a million neurons must utilize efficient wiring and neural algorithms to perceive and act in the world, the tiny AI systems powering tomorrow's edge computing devices will require clever, efficient solutions to operate with minimal power and footprint," explains Olshausen. Remarkably, the efficiency of KWS is such that it can be deployed on hardware costing as little as $0.10.
Gert Cauwenberghs, a professor at UC San Diego and Co-Director of the Institute for Neural Computation, highlights the significance of efficient AI models in the context of wearable brain and body-sensing technology.
"Making sense of the massive data streaming from these sensors despite the high levels of variability and noise in their biological operating environments is a major challenge that calls for powerful AI, down to the physiological interface," notes Cauwenberghs. By leveraging Aizip's ADA tools, Cauwenberghs's group collaborates with Aizip to demonstrate efficient AI models for in-ear electrophysiological brain-state monitoring.
To produce AI models at scale for these applications, ADA is indispensable. Yubei Chen, CTO of Aizip and a professor at UC Davis, envisions the creation of an "AI Nanofactory"—Aizipline. Chen emphasizes the need to simplify each stage and develop ADA tools accordingly. "By leveraging foundation, visual-language, and generative AI models, the data collection, preparation, and testing process can be accelerated by orders of magnitude," Chen says.
"Leveraging self-supervised learning, efficient architecture space, hardware-aware architecture search, scalable compilation, and many other techniques, our model design and development cycle can be further shrunk significantly." Over the past two years, AI-design pipeline efficiency at Aizip has increased more than tenfold.
As Aizip advances its automated AI-design paradigm, it grapples with fundamental questions that arise along the way. "How do we find the right data among massive data? How to generate data from limited data? How to define and ensure real-world robustness? How to maximize the capacity of a neural network under resource constraints? How to debug an AI model?" ponders Chen. Aizip's relentless pursuit is to simplify and optimize every stage of AI design, with minimal human intervention.
Yan Sun, Chairman and CEO of Aizip, underscores the importance of customized and efficient AI in real-world applications. With the aid of AI tools, Aizip has developed and tested tens of thousands of models, delivering hundreds of them to customers worldwide. These models now support volume shipping in both consumer and enterprise markets, driving societal benefits and promoting sustainability. Aizip remains steadfast in its commitment to bringing pervasive AI to every aspect of life, anywhere and at any time.
As we witness this revolution unfold, Aizip's commitment to pushing the boundaries of AI innovation promises a brighter and more efficient future for us all.
For more science and technology stories check out our New Innovations section at The Brighter Side of News.
Note: Materials provided above by The Brighter Side of News. Content may be edited for style and length.
Like these kind of feel good stories? Get the Brighter Side of News' newsletter.
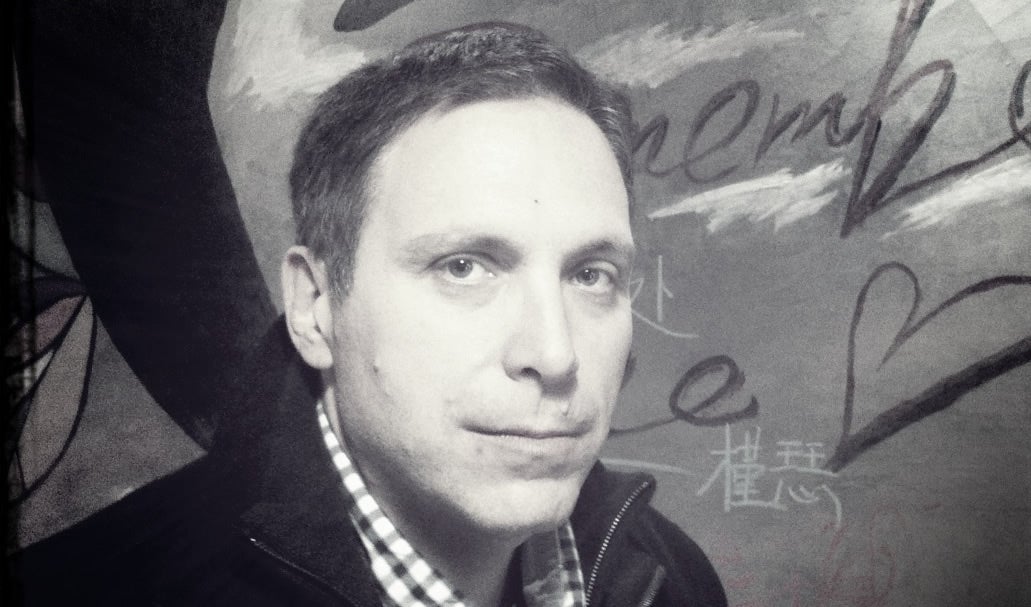