Cracking the Code: AI Solves Equation for Galaxy ‘Weight’
The astrophysicists noted that these improved mass estimates will assist in determining the fundamental properties of the universe.
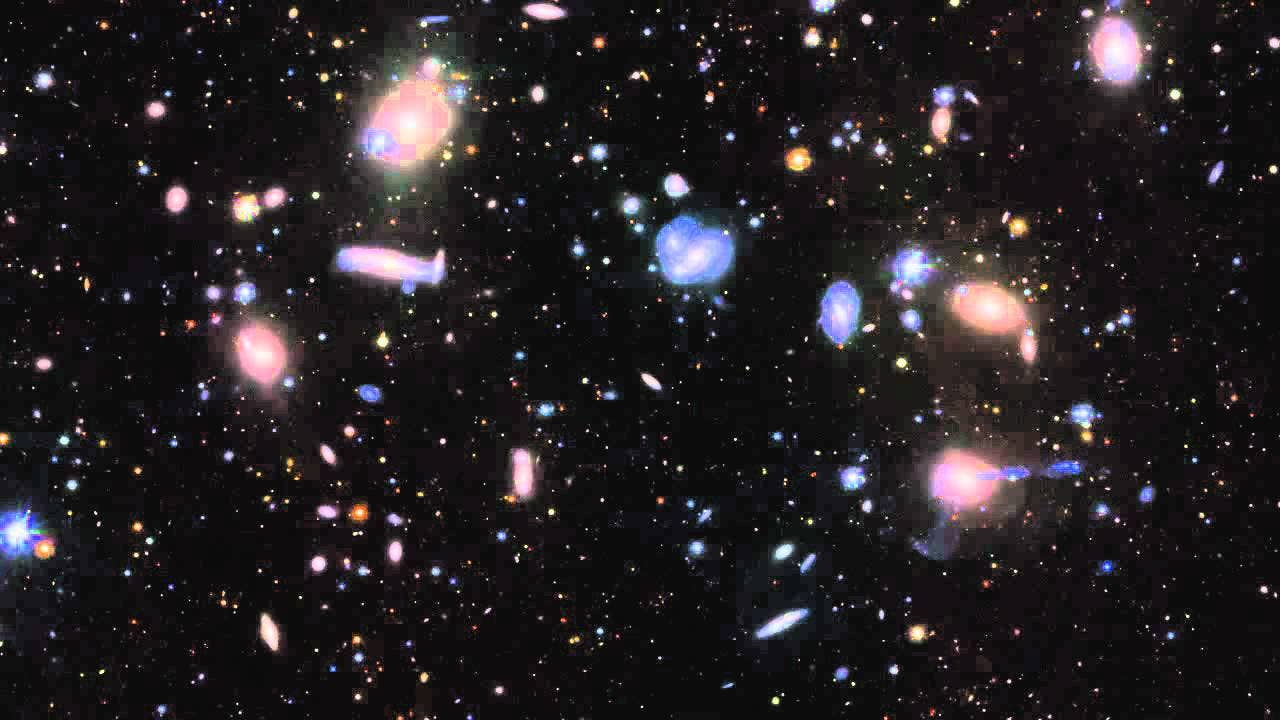
[Mar. 24, 2023: JJ Shavit, The Brighter Side of News]
Interacting Galaxies In The Hercules Cluster. (CREDIT: The VLT Survey Telescope (VST) at ESO's Paranal Observatory in Chile)
Astrophysicists from the Institute for Advanced Study, the Flatiron Institute, and their colleagues have utilized artificial intelligence to enhance the method of estimating the mass of colossal galaxy clusters. By adding a simple term to an existing equation, the AI has enabled scientists to produce more accurate mass estimates than they had previously.
The astrophysicists noted that these improved mass estimates will assist in determining the fundamental properties of the universe with greater accuracy. The findings were published in the Proceedings of the National Academy of Sciences.
Francisco Villaescusa-Navarro, a research scientist at the Center for Computational Astrophysics (CCA) at the Flatiron Institute in New York City and a co-author of the study, commented on the simplicity of the discovery. He stated that nobody had previously discovered the term, despite scientists having worked on the subject for decades.
The research was led by Digvijay Wadekar from the Institute for Advanced Study in Princeton, New Jersey, and involved scientists from the CCA, Princeton University, Cornell University, and the Center for Astrophysics | Harvard & Smithsonian.
Related Stories
To understand the universe, one must determine the location and amount of matter in it. Galaxy clusters are the universe's most massive objects and can contain hundreds to thousands of galaxies, as well as hot gas, plasma, and dark matter. These clusters' gravity holds their components together, making it crucial to comprehend their properties to understand the universe's origin and evolution.
Measuring the total mass of a galaxy cluster is challenging because galaxies cannot be weighed like ordinary objects. Moreover, the dark matter that comprises a significant portion of a cluster's mass is invisible. Scientists estimate the mass of a cluster by deducing it from observable quantities.
Rashid Sunyaev and Yakov B. Zel'dovich developed a new method to estimate galaxy cluster masses in the early 1970s. Their approach relies on measuring the properties of photons generated by the interaction of photons from the Big Bang's afterglow with electrons in squeezed matter. This interaction alters the electrons' interaction with photons based on the strength of gravity compressing the material, which depends on the cluster's mass. Astrophysicists can then estimate the cluster's mass by analyzing the properties of the photons.
This image taken by NASA's Hubble Space Telescope shows a spiral galaxy (bottom left) in front of a large galaxy cluster. New research leveraged an artificial tool to estimate the masses of galaxy clusters more accurately. (CREDIT: ESA/Hubble & NASA)
Symbolic regression, an artificial intelligence tool, was employed by Wadekar and his colleagues to improve the estimation of galaxy cluster mass. The tool attempts to find a better approach by testing various combinations of mathematical operators, such as addition and subtraction, with different variables, to determine the equation that best matches the data. However, the "integrated electron pressure" remains an imperfect proxy for mass, as photon property changes differ depending on the cluster.
Wadekar and his team utilized an advanced universe simulation containing numerous galaxy clusters as input for their AI program. The program, developed by CCA research fellow Miles Cranmer, then searched for additional variables that could enhance the accuracy of mass estimations.
AI is highly valuable in identifying parameter combinations that may be overlooked by human analysts. While humans may easily identify a couple of significant parameters, AI can efficiently parse through vast amounts of data, often uncovering unexpected influencing factors.
According to Wadekar, while deep neural networks are powerful, they are often like black boxes, making it challenging to comprehend their inner workings. In contrast, symbolic regression is beneficial in that it generates simple mathematical expressions in the form of easy-to-understand equations, providing an interpretable model.
The researchers were able to improve the accuracy of galaxy cluster mass estimations by incorporating a single new term into the existing equation, thanks to the symbolic regression program. The AI-generated equation prompted the team to look further into gas concentration and its correlation with the less reliable regions of galaxy clusters, such as the cores of galaxies where supermassive black holes are present. The new equation downplays the importance of these complex cores, resulting in more reliable mass inferences by focusing on the doughy outskirts of the galaxy clusters, similar to a spherical doughnut.
Thousands of simulated universes from the CCA's CAMELS suite were used to test the equation discovered by AI researchers. The equation was found to reduce the variability in mass estimates of large galaxy clusters by approximately 20 to 30 percent, compared to the currently used equation.
Using SR on the data from the IllustrisTNG hydrodynamical simulation, we find a new proxy for cluster mass which combines YSZ and concentration of ionized gas (cgas): M ∝ Yconc3/5 ≡ YSZ3/5(1 − A cgas). Yconc reduces the scatter in the predicted M by ∼20 − 30% for large clusters (M ≳ 1014 h−1 M⊙), as compared to using just YSZ. (CREDIT: PNAS)
With the new equation, observational astronomers engaged in upcoming galaxy cluster surveys can gain better insights into the mass of the observed objects. The Simons Observatory, the Stage 4 CMB experiment, and the eROSITA X-ray survey are among the many planned surveys targeting galaxy clusters. The new equation can help maximize the scientific return from these surveys.
According to Wadekar, this publication is just the beginning of using symbolic regression in astrophysics. Symbolic regression and other artificial intelligence tools can help answer various astrophysical questions, from studying small astrophysical systems such as exoplanets to investigating galaxy clusters, which are the biggest things in the universe. In astronomy, it is common for people to make a linear fit between two parameters and ignore everything else, but these tools enable us to go beyond existing two-parameter power laws.
Note: Materials provided above by The Brighter Side of News. Content may be edited for style and length.
Like these kind of feel good stories? Get the Brighter Side of News' newsletter.
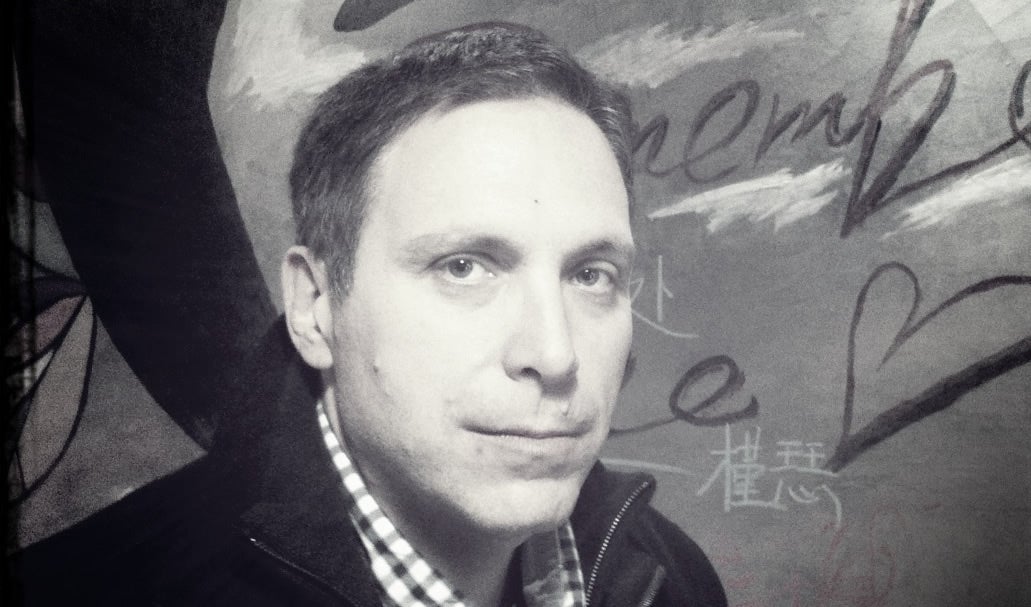