Cutting-edge AI system predicts changes in water quality before they happen
Scientists use AI and real-time stream data to predict turbidity, offering water systems new tools to prepare for threats before they strike.
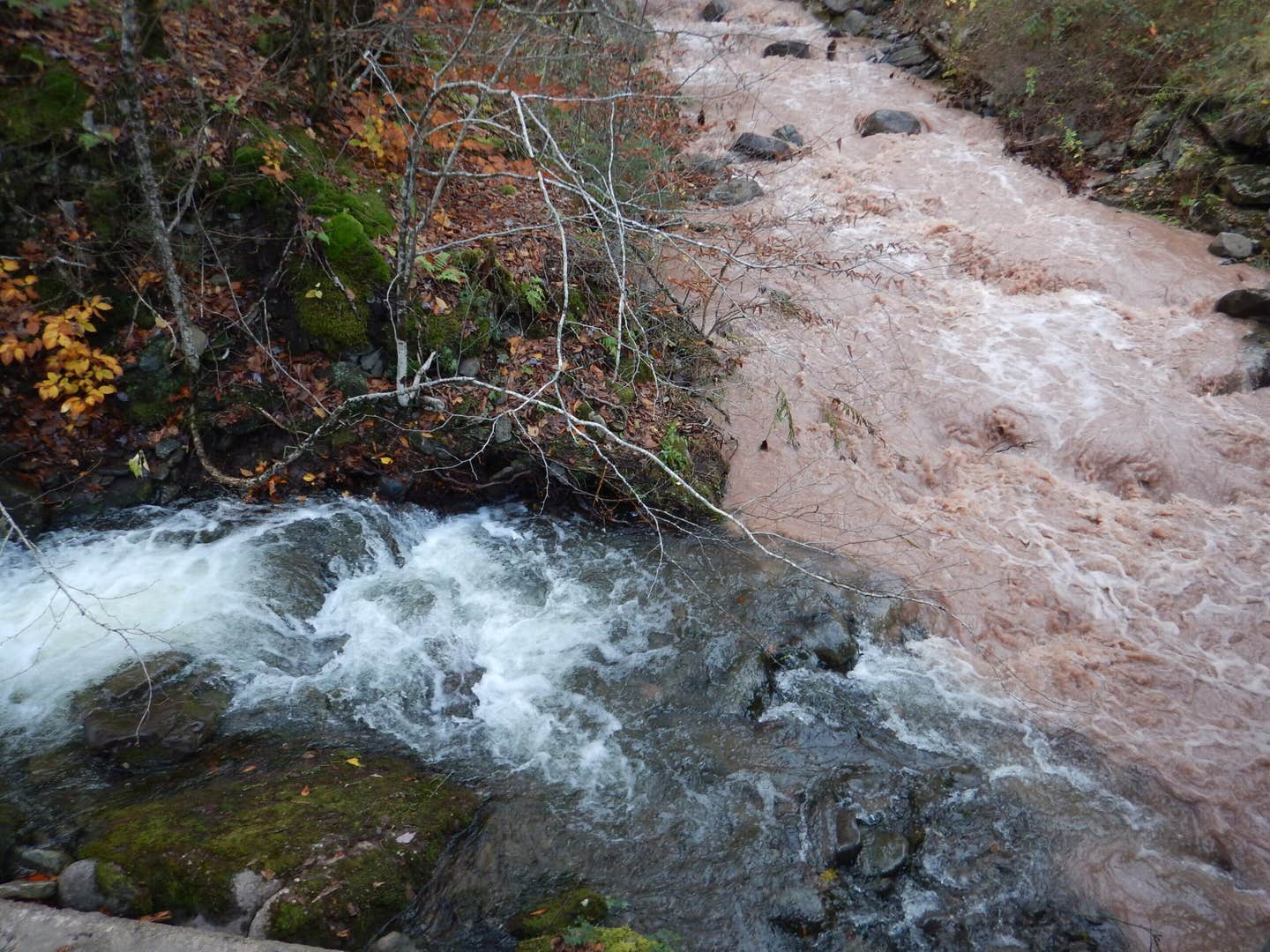
AI and streamflow models combine to forecast water quality, helping communities predict turbidity and manage safe drinking water. (CREDIT: Dany Davis)
The future of clean drinking water may depend on how well science can forecast not just how water moves—but also what’s in it. A powerful combination of artificial intelligence, high-frequency sensor data, and advanced streamflow models now allows researchers to predict changes in water quality before they happen.
This advance could help water systems nationwide stay ahead of threats like sediment spikes after storms, harmful algal blooms, or fertilizer runoff. A group of researchers from the University of Vermont has taken the first steps to prove that predicting water quality, not just water quantity, is now possible.
Turning Flow Models into Water Quality Tools
Across the country, scientists and water managers already rely on the National Water Model (NWM), a large-scale computer system built to predict how streams and rivers will flow. It uses weather forecasts, rainfall data, and stream observations to estimate how water will move across the landscape. These predictions help people prepare for flooding, droughts, and other extreme events.
Now, researchers are transforming the model's abilities. By adding machine learning and sensor data, they’ve created a system that can also forecast turbidity, which measures how cloudy or clear water is due to sediment and particles.
Scientist Andrew Schroth led the study. “This new tool can be implemented across the country and broadly utilized by folks that could use water quality forecasts in any number of applications,” he said. In a first-of-its-kind move, his team showed that by combining AI with the NWM, they could predict when water becomes murky—days in advance.
The Test Site: Esopus Creek
To prove their idea worked, the team chose a water system with real-world challenges: New York State’s Esopus Creek, part of the Catskill Mountains. This creek flows into the Ashokan Reservoir, which supplies nearly 40% of New York City’s drinking water. The city relies on this reservoir as part of the country’s largest unfiltered water system.
Related Stories
Turbidity is one of the biggest concerns for this water source. When it rises too high—usually during or after storms—the city must reduce the reservoir’s use. That shift affects water delivery, planning, and costs.
The problem starts in the land itself. The Esopus Creek valley holds a lot of loose glacial sediment, including fine clay, silt, and gravel. When storms hit, the streambanks erode, washing these particles into the creek. The water becomes cloudy. This turbidity can linger for weeks or even months.
“When too much sediment comes into the reservoir during or after big storms, New York City has to limit supply and modify their operations,” Schroth said. That’s why his team focused on predicting turbidity, not just flow.
How Machine Learning Improves Forecasts
The researchers built their forecast tool by training a machine learning model called LightGBM. This AI system uses data from over five years of sensor readings that monitor turbidity and streamflow every few minutes.
They fed the model forecasts from the NWM and trained it to learn how future streamflow patterns lead to changes in turbidity. The model then predicted turbidity levels up to three days in advance with high accuracy.
Compared to simpler models, the machine learning version performed better. It handled complex landscapes more easily and produced reliable results, especially for short-term forecasts. It even showed which conditions—like high water flow—most affected turbidity.
This finding is important. It shows that clear and efficient AI tools, when combined with sensor data and streamflow forecasts, can help predict water quality. The study marks the first time that the NWM has been used in this way.
John Kemper, a scientist who worked on the study, highlighted the value of the project. “Turning a streamflow forecasting tool into a water quality forecasting tool paves the way for increasingly available forecasts to serve community needs,” he said.
A Model for the Whole Country
The success in the Catskills shows how the model could be useful far beyond New York. Supported by the Cooperative Institute for Research to Operations in Hydrology (CIROH), a national partnership funded by NOAA and the U.S. Geological Survey, the researchers are now working to expand the tool’s reach.
Across the United States, hundreds of communities face water quality challenges. Many use sensors to monitor turbidity, nutrients, and pollutants. Now, with a forecasting tool like this, they could get early warnings about incoming problems.
For example, a water treatment plant could use turbidity forecasts to prepare for incoming sediment, possibly avoiding a shutdown. A local health department could respond faster to potential algal blooms, closing beaches before swimmers get sick. Farmers could adjust fertilizer use based on expected water levels, avoiding runoff that harms rivers and lakes.
The model works by identifying links between water flow and specific pollutants or conditions. In New York, it focused on turbidity. But in other places, it could target nutrients like nitrogen or phosphorus, or other substances like chloride. The structure of the model allows it to be adapted to different needs.
“This opens a new window that can really benefit the country as a whole moving forward,” Schroth said.
Ready for Real-World Impact
The system doesn’t just work in theory. The National Water Model already provides hourly streamflow forecasts across the U.S. These are public and easy to access. The sensors many water systems use also deliver frequent updates. By combining the two and training local AI models, communities can start building their own water quality forecasts.
The work shows that data science and environmental science can come together to solve urgent problems. As climate change increases the number and intensity of storms, sediment and pollution risks will rise too. Being able to predict these events could help reduce health risks, protect ecosystems, and save money on water treatment.
This project proves that with the right tools, even small research teams can deliver big results. With continued support, such models could become a core part of how the country manages its water resources in the years ahead.
Research findings are available online in the Journal of the American Water Resources Association.
Note: The article above provided above by The Brighter Side of News.
Like these kind of feel good stories? Get The Brighter Side of News' newsletter.
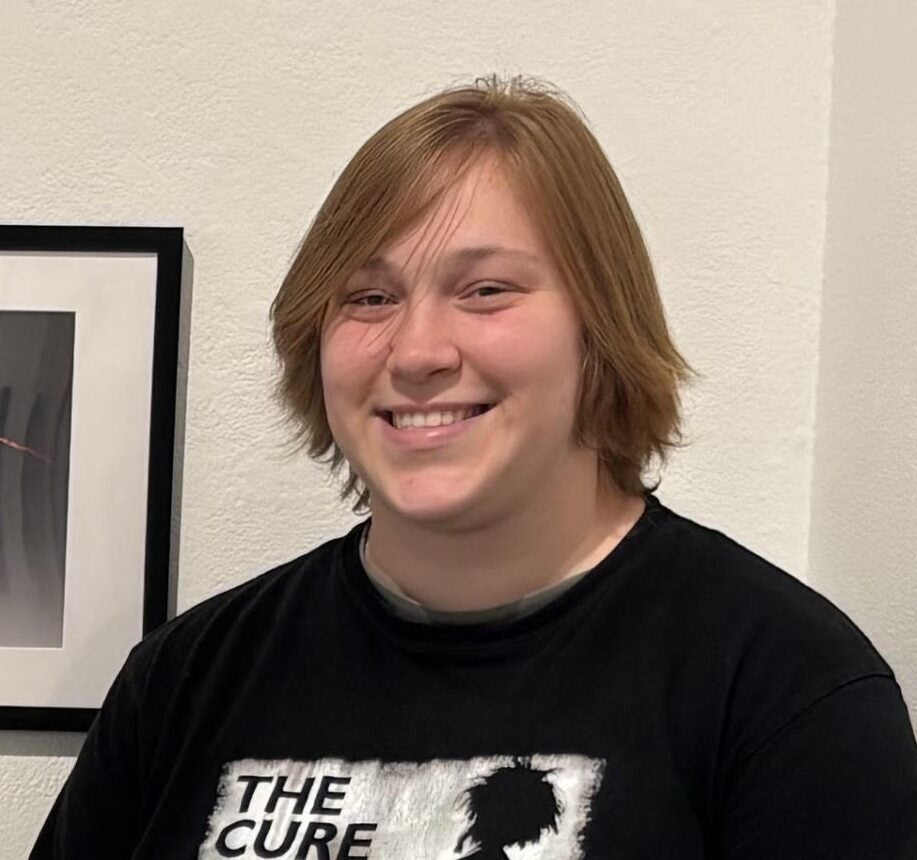
Mac Oliveau
Science & Technology Writer | AI and Robotics Reporter
Mac Oliveau is a Los Angeles–based science and technology journalist for The Brighter Side of News, an online publication focused on uplifting, transformative stories from around the globe. Passionate about spotlighting groundbreaking discoveries and innovations, Mac covers a broad spectrum of topics—from medical breakthroughs and artificial intelligence to green tech and archeology. With a talent for making complex science clear and compelling, they connect readers to the advancements shaping a brighter, more hopeful future.