Data scientists predict stock performance using AI and online news
Their method, far exceeds the accuracy of traditional models, is innovative and provides insights into the heart of machine learning.
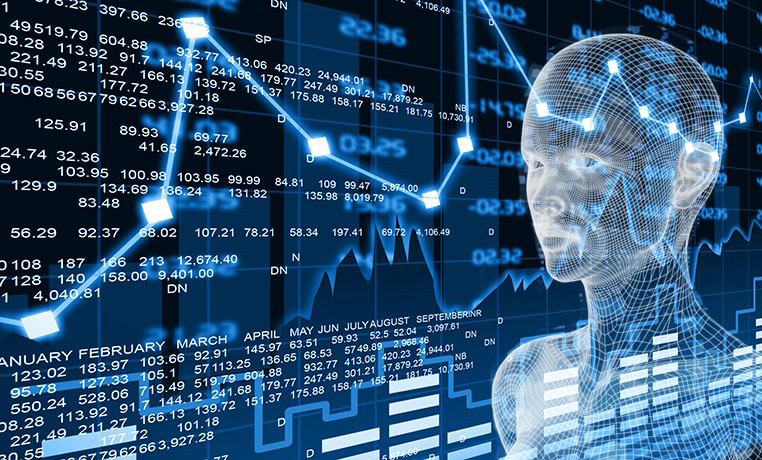
[July 15, 2023: Staff Writer, The Brighter Side of News]
Researchers developed a "nano-excitonic transistor" using intralayer and interlayer excitons in heterostructure-based semiconductors. (CREDIT: Creative Commons)
By harnessing the power of interdisciplinary fields such as machine learning, natural language processing (NLP), and finance, a team of Cornell researchers have unlocked a new avenue for predicting financial returns. Their method, far exceeding the accuracy of traditional models, is not only innovative but also provides insights into the heart of machine learning, a domain often considered inscrutable.
In their groundbreaking paper, "News-Based Sparse Machine Learning Models for Adaptive Asset Pricing," published in the journal Data Science in Science, the researchers shed light on a new, intelligible machine-learning framework that incorporates stock- and industry-specific information garnered from financial news. This bold and innovative approach is poised to redefine the landscape of financial forecasting.
“The criticism often leveled against machine learning is its lack of interpretability,” shared Martin Wells, the Charles A. Alexander Professor of Statistical Sciences in the Cornell Ann. S Bowers College of Computing and Information Science and the paper’s senior author. He went on to explain that, “Frequently, researchers utilizing large-scale models might grapple with understanding what the outputs mean or identifying what underpins the model. Our research flips the script. We harness text data from news to forge interpretable machine-learning models that unveil important features explicitly."
The team's approach capitalizes on the text data's power to "cluster the data," bringing a semblance of order to the typically chaotic results produced by algorithms. This idea was elucidated by the paper's lead author, Liao Zhu, Ph.D. ’20, who embarked on a career in the finance industry after completing the paper. He said, “Our hypothesis contends that the financial news can potentially enhance our comprehension of the types of stocks associated with certain tradable assets.”
Related Stories
Zhu elaborated that these assets could encompass exchange-traded funds (ETFs), essentially a collection of stocks tracking an entire sector.
The research, a natural progression of Zhu's earlier work initiated during his doctoral studies under Wells and Robert Jarrow, the Ronald P. & Susan E. Lynch Professor of Investment Management at the Samuel Curtis Johnson Graduate School of Management, also features contributions from Peter (Haoxuan) Wu, Ph.D. ’23.
The usage of traditional statistical methods to sift through market data to elucidate stock returns is not a novel concept. Likewise, the application of text data, more specifically sentiment analysis—a subfield of NLP, to mine the Internet for positive or negative words linked to a company that could potentially influence a stock's trajectory, is not new.
Visualization of asset embedding vectors. Here we use UMAP to project them to 2-dimension only for visualization. The ETFs clustering in the NEUSS algorithm uses a different UMAP. (CREDIT: Data Science)
However, the Cornell researchers' approach pioneers new territory by proposing a dynamic prediction framework that integrates market data and text data without sentiment analysis. This method involves borrowing the "word embeddings" concept from NLP to create "asset embeddings" for a specific set of tradable assets, using an algorithm to process financial news. After converting both text and market data into numerical form, custom-designed algorithms are unleashed to process these figures.
Zhu elucidated their unique approach, stating, “Our algorithm eschews the sentiment derived from the news and instead utilizes the news as a guide for which assets or words should be considered for each particular stock or industry. This reveals more stock- and industry-specific information.”
Adjusted R-Squared for explanation for FF5 vs NEUSS model. The plot illustrate the density adjusted R-squared for FF5 vs NEUSS for in-sample explanation and out-of-sample explanation comparisons. As seen, NEUSS outperforms FF5 in both in-sample and out-of-sample explanation. (a) In-sample Adjusted R-squared. (b) Out-of-sample Adjusted R-squared. (CREDIT: Data Science)
The research team compiled an enormous corpus of online financial news articles spanning 2013 to 2019 and fed this data to their algorithm. The machine learning model then began associating specific assets and words with certain stocks and industries. Armed with an AI-optimized language map, the researchers gained a deeper understanding of which specific assets and words merited consideration.
The application of this methodology led to the development of two distinct models. The News Embedding UMAP Sparse Selection (NEUSS) model generates predictions for individual stock returns, while the News Sparse Encoder with Rationale (INSER) model identifies key words relevant to each specific industry before utilizing them to predict industry returns more precisely.
Number of basis assets selected for explanations. The plot illustrates histogram of count of stocks with varying number of basis assets selected for explaining returns. As seen, the majority of stocks require less than 5 basis assets for explanation, which indicates the overfit of the FF5 model. (CREDIT: Data Science)
For instance, the NEUSS model may determine that an exchange-traded fund tracking the semiconductor manufacturing sector is valuable in predicting the stock returns of a specific tech company, but not applicable to predict returns of stocks in other sectors such as retail or wholesale. Similarly, the INSER model might identify the term “plant” as significant for the energy industry, but irrelevant for others, like social media.
The interdisciplinary, interpretative approach proved to be successful. The NEUSS model surpassed the traditional predictive benchmark—known as the Fama-French 5-factor model—by 50%, while the INSER model outperformed its benchmark (without industry-specific information) by 10%.
These advancements signal a revolution in the finance field, with complex machine-learning algorithms and diverse data types driving change. According to Zhu and Wells, the transformation is already underway.
Zhu confidently declared, “I believe the AI revolution in finance is already here,” adding, “and our paper is propelling an aspect of that revolution forward.” As this groundbreaking research continues to unfold, the future of financial prediction models and the use of AI in finance will undoubtedly be redefined.
For more science and technology news stories check out our New Innovations section at The Brighter Side of News.
Note: Materials provided above by The Brighter Side of News. Content may be edited for style and length.
Like these kind of feel good stories? Get the Brighter Side of News' newsletter.
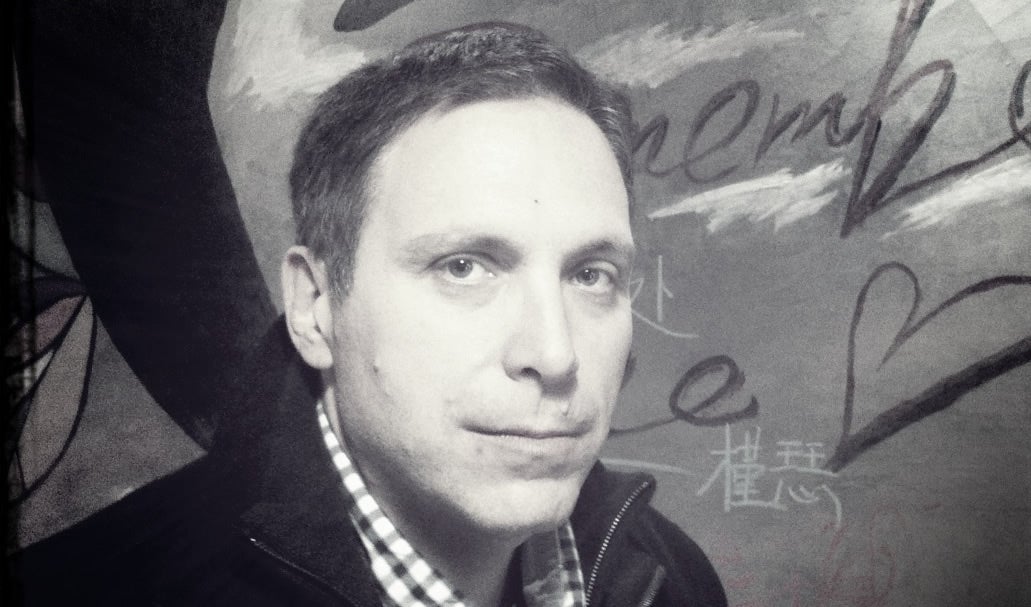
Joseph Shavit
Head Science News Writer | Communicating Innovation & Discovery
Based in Los Angeles, Joseph Shavit is an accomplished science journalist, head science news writer and co-founder at The Brighter Side of News, where he translates cutting-edge discoveries into compelling stories for a broad audience. With a strong background spanning science, business, product management, media leadership, and entrepreneurship, Joseph brings a unique perspective to science communication. His expertise allows him to uncover the intersection of technological advancements and market potential, shedding light on how groundbreaking research evolves into transformative products and industries.