Game-changing wrist-mounted camera captures entire body in 3D
Using a miniature camera and a customized deep neural network, researchers have developed a first-of-its-kind wristband that tracks posture.
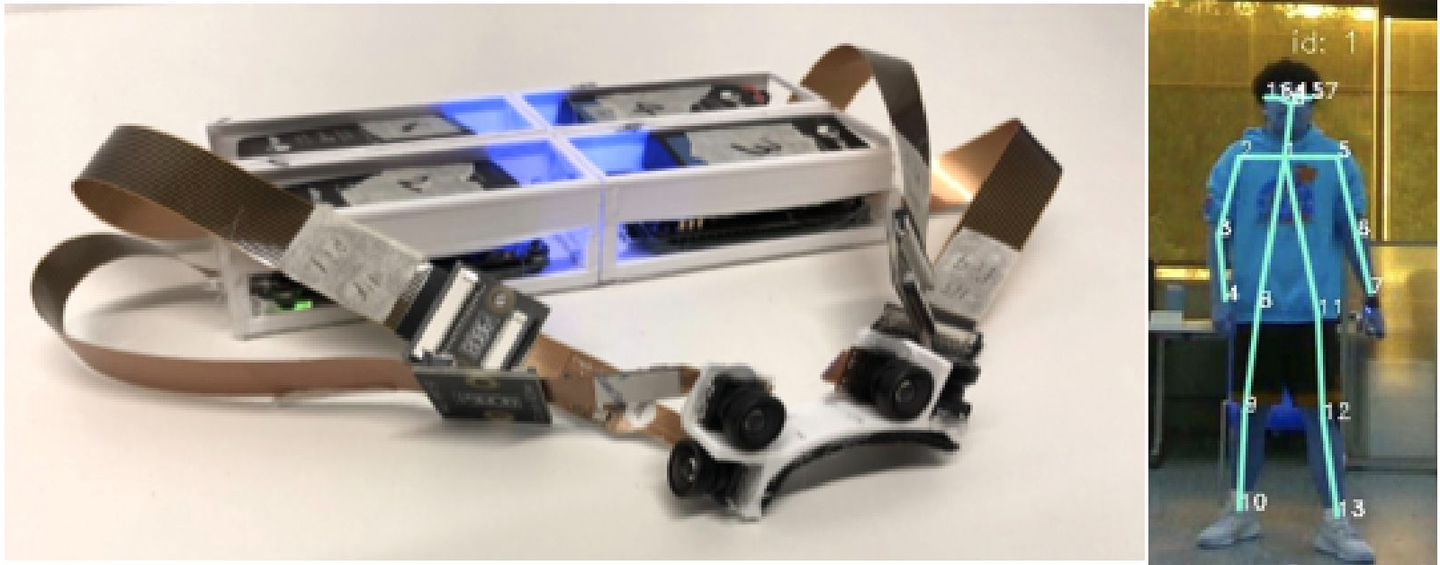
[Nov. 10, 2022: Becka Bowyer, Cornell University]
BodyTrak, an intelligent sensing technology that can estimate full body poses on a wristband. It only requires one miniature RGB camera to capture the body silhouettes. (CREDIT: Proceedings of the Association for Computing Machinery on Interactive, Mobile, Wearable and Ubiquitous Technologies)
Body pose estimation is becoming increasingly important in many fields such as health (e.g., physiology of individuals with physical disorders such as scoliosis and Parkinson’s), the gaming industry, sports analysis, and even communication studies which can help us understand how we interact with one another through our body language.
Previous research has used methods such as external sensors placed within a room, depth cameras. It works for some applications, e.g., motion games at home. However, these solutions are limited in terms of mobility, not allowing
reconstructing the body in the field.
To address these mobility issues, researchers have used wearable solutions to estimate body poses. Most of these systems require the users to wear multiple sensors (e.g., IMU) on the body, which are less practical in the realworld setting. The recent advancements show that using a single form factor, such as a chest-mounted camera, a handheld smartphone or a hat-mounted camera, can also estimate full-body poses with encouraging performances.
However, these form factors (chest-mount or hat) may not be immediately acceptable or convenient for users to be worn in different daily activities. For instance, chest-mounted devices such as GoPro are acceptable for a group of users in specific contexts. However, it is still not yet be acceptable to be worn on a daily basis for many. Therefore, in the future eco-system of wearables, it is essential to offer users a variety of wearable sensing technologies to track body poses to decide the technology based on the context.
Related News
Using a miniature camera and a customized deep neural network, Cornell researchers have developed a first-of-its-kind wristband that tracks the entire body posture in 3D.
BodyTrak is the first wearable to track the full body pose with a single camera. If integrated into future smartwatches, BodyTrak could be a game-changer in monitoring user body mechanics in physical activities where precision is critical, said Cheng Zhang, assistant professor of information science and the paper’s senior author.
“Since smartwatches already have a camera, technology like BodyTrak could understand the user’s pose and give real-time feedback,” Zhang said. “That’s handy, affordable and does not limit the user’s moving area.”
The full prototype consists of the wristband, the Intel RealSense Depth Camera, and a PC. When setting up the prototype for data collection, the cameras are mounted onto the velcro strap which is then wrapped around the participant’s wrist. The participant then holds the 3D box which holds the raspberry pi boards and the accompanying power sources. (CREDIT: BodyTrak: Inferring Full-body Poses from Body Silhouettes using a Wristband)
A corresponding paper, “BodyTrak: Inferring Full-body Poses from Body Silhouettes Using a Miniature Camera on a Wristband,” was published in the Proceedings of the Association for Computing Machinery (ACM) on Interactive, Mobile, Wearable and Ubiquitous Technology, and presented in September at UbiComp 2022, the ACM international conference on pervasive and ubiquitous computing.
BodyTrak is the latest body-sensing system from the SciFiLab – based in the Cornell Ann S. Bowers College of Computing and Information Science – a group that has previously developed and leveraged similar deep learning models to track hand and finger movements, facial expressions and even silent-speech recognition.
Design Space. A matrix of 12 body movements that cover the full range of body movements. (CREDIT: BodyTrak: Inferring Full-body Poses from Body Silhouettes using a Wristband)
The secret to BodyTrak is not only in the dime-sized camera on the wrist, but also the customized deep neural network behind it. This deep neural network – a method of AI that trains computers to learn from mistakes – reads the camera’s rudimentary images or “silhouettes” of the user’s body in motion and virtually re-creates 14 body poses in 3D and in real time.
In other words, the model accurately fills out and completes the partial images captured by the camera, said Hyunchul Lim, a doctoral student in the field of information science and the paper’s lead author.
Ground Truth Acquisition. Image (a) represents the ground truth that is displayed when using the RealSense depth camera. In image, (b) we depict the normalization values for each body part. In figure (c) the image displays the normalized skeleton after it is passed through the deep learning pipeline. (CREDIT: BodyTrak: Inferring Full-body Poses from Body Silhouettes using a Wristband)
“Our research shows that we don’t need our body frames to be fully within camera view for body sensing,” Lim said. “If we are able to capture just a part of our bodies, that is a lot of information to infer to reconstruct the full body.”
Maintaining privacy for bystanders near someone wearing such a sensing device is a legitimate concern when developing these technologies, Zhang and Lim said. They said BodyTrak mitigates privacy concerns for bystanders since the camera is pointed toward the user’s body and collects only partial body images of the user.
Camera Setting. In this figure is the camera arrangement, along with their angular measurements. The x plane runs horizontally across the wrist, the y plane runs vertically across the wrist, and the z plane runs away from the wrist. (CREDIT: BodyTrak: Inferring Full-body Poses from Body Silhouettes using a Wristband)
They also recognize that today’s smartwatches don’t yet have small or powerful enough cameras and adequate battery life to integrate full body sensing, but could in the future.
Along with Lim and Zhang, paper co-authors are Matthew Dressa ’22, Jae Hoon Kim ’23 and Ruidong Zhang, a doctoral student in the field of information science; Yaxuan Li of McGill University; and Fang Hu of Shanghai Jian Tong University.
Note: Materials provided above by Cornell University. Content may be edited for style and length.
Like these kind of feel good stories? Get the Brighter Side of News' newsletter.
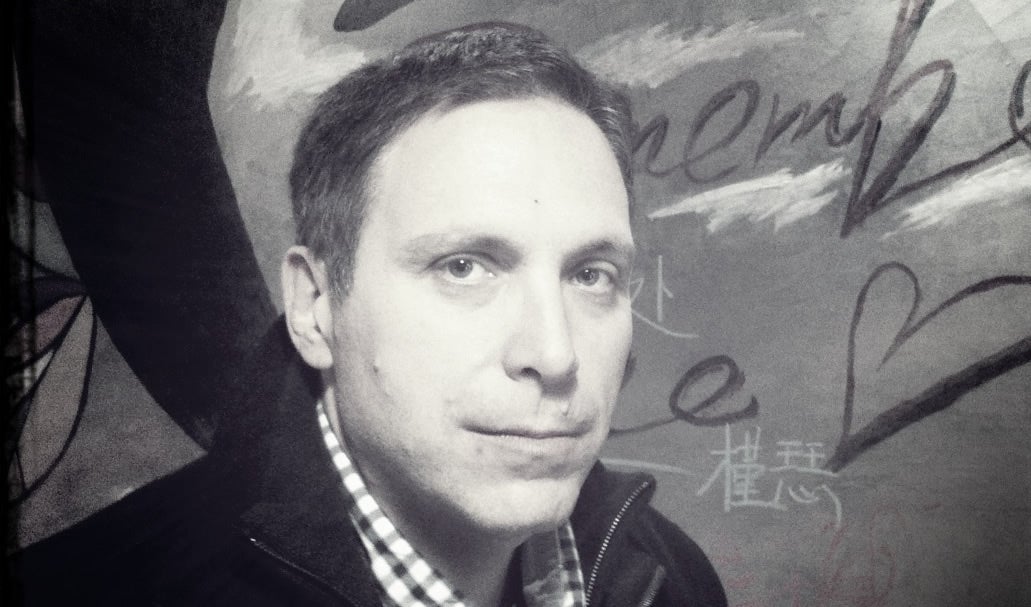