Groundbreaking AI-powered system designs novel cancer drug in 30 days
The drug has the potential to be a new therapy for a variety of cancers, including breast, lung, and pancreatic cancer.
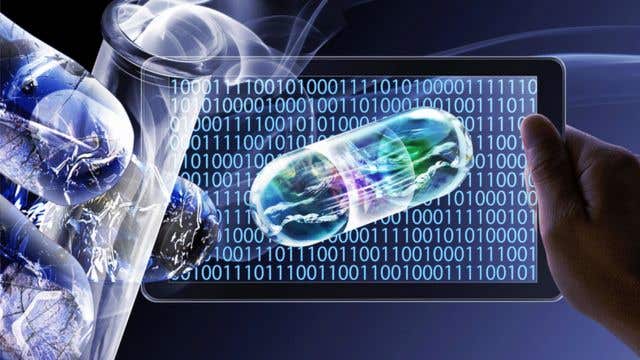
[Mar. 21, 2023: JD Shavit, The Brighter Side of News]
Researchers believe that the drug has the potential to be a new therapy for a variety of cancers, including breast, lung, and pancreatic cancer. (CREDIT: Creative Commons)
Artificial intelligence (AI) is revolutionizing many fields, including drug discovery. With the ability to process vast amounts of data and analyze complex patterns, AI is proving to be a valuable tool in identifying potential new drugs for a variety of diseases, including cancer.
Researchers at the University of Toronto have recently used an AI-powered database to design a potential cancer drug in just 30 days. The team, led by Professor Igor Stagljar and Dr. Reza Salavati, used a machine learning algorithm to analyze data from over 1.4 million compounds and predict which ones would be effective in blocking the activity of a protein called MTH1, which is critical for cancer cell survival.
The study, which was published in the journal Nature Communications, represents a significant breakthrough in the field of drug discovery. Traditionally, the drug discovery process is slow and costly, with a high failure rate. It can take years, if not decades, to develop a new drug from the initial discovery phase to clinical trials. However, by using AI to streamline the process, researchers can accelerate drug discovery and potentially bring new treatments to patients more quickly.
The researchers at the University of Toronto started by creating a database of over 1.4 million compounds, which they then screened using a machine learning algorithm. The algorithm was trained on data from previous studies of MTH1 inhibitors, allowing it to identify compounds that were likely to be effective based on their chemical structure and other properties.
Related Stories:
Once the algorithm had identified a set of promising compounds, the researchers used a technique called protein-ligand docking to assess how well the compounds would bind to MTH1. Protein-ligand docking is a computational method that predicts the binding affinity of a small molecule (the ligand) to a protein target. By using this technique, the researchers were able to identify compounds that would be most likely to block the activity of MTH1.
The team then synthesized the top 20 compounds and tested them in vitro, or in a laboratory setting, to determine their effectiveness in blocking MTH1 activity. They found that one compound in particular, which they called TH5427, was highly effective in inhibiting MTH1 and had the potential to be developed into a new cancer drug.
TH5427 works by blocking the activity of MTH1, which is critical for cancer cell survival. MTH1 is an enzyme that helps to protect cancer cells from the damage caused by oxidative stress. Cancer cells produce high levels of reactive oxygen species (ROS), which can damage DNA and other cellular components. MTH1 helps to remove damaged nucleotides from the cell, preventing them from being incorporated into DNA and causing further damage.
Machine learning algorithm analyzes data from over 1.4 million compounds and predicts which ones would be effective in blocking the activity of a protein called MTH1, which is critical for cancer cell survival. (CREDIT: Insilico Medicine)
By blocking MTH1, TH5427 prevents cancer cells from repairing the damage caused by ROS, leading to their death. Importantly, TH5427 appears to be highly selective for cancer cells, meaning that it does not affect normal cells in the body. This is a key feature of any potential cancer drug, as it minimizes the risk of side effects.
The researchers also tested TH5427 in mouse models of cancer and found that it was highly effective in slowing tumor growth. They believe that the drug has the potential to be developed into a new therapy for a variety of cancers, including breast, lung, and pancreatic cancer.
This work is the first demonstration of applying AlphaFold to the hit identification process in drug discovery. (CREDIT: Nature Communications)
The success of this study highlights the potential of AI in drug discovery. By using machine learning algorithms to analyze large datasets and predict which compounds are most likely to be effective, researchers can accelerate the drug discovery process and potentially bring new treatments to patients more quickly. This is particularly important for diseases like cancer, where time is of the essence and patients need effective treatments as soon as possible.
However, there are also some challenges to using AI in drug discovery. One of the biggest challenges is the quality of the data that is used to train the algorithms. In order for machine learning algorithms to be effective, they need to be trained on high-quality data that is both accurate and representative of the real-world situation. In the case of drug discovery, this can be challenging, as there may be biases or gaps in the available data that can affect the accuracy of the algorithm's predictions.
Another challenge is the interpretation of the results. While machine learning algorithms can identify patterns and make predictions based on large datasets, they are not always able to explain why a particular compound or approach is effective. This can make it difficult for researchers to understand the underlying mechanisms of the drug's action and to optimize its effectiveness.
Despite these challenges, the potential of AI in drug discovery is clear. In addition to accelerating the drug discovery process, AI can also help to identify new targets for drug development and to optimize existing drugs to make them more effective.
The success of the University of Toronto study has also inspired other researchers to explore the use of AI in drug discovery. For example, a team of researchers at MIT recently used AI to design a new antibiotic that was effective against a wide range of bacteria, including some that are resistant to existing antibiotics. The researchers used a machine learning algorithm to analyze data from over 6,000 compounds and identify those that were most likely to be effective against certain types of bacteria.
Another example comes from Insilico Medicine, a company that is using AI to identify potential new drugs for a variety of diseases, including cancer, Alzheimer's disease, and Parkinson's disease. The company has developed a platform called GENTRL, which uses deep learning algorithms to generate new compounds that are optimized for specific targets.
As the use of AI in drug discovery continues to grow, it is likely that we will see more breakthroughs like the one achieved by the University of Toronto researchers. By combining the power of machine learning with the expertise of drug discovery experts, we may be able to develop new treatments for some of the world's most pressing health challenges.
Note: Materials provided above by The Brighter Side of News. Content may be edited for style and length.
Like these kind of feel good stories? Get the Brighter Side of News' newsletter.
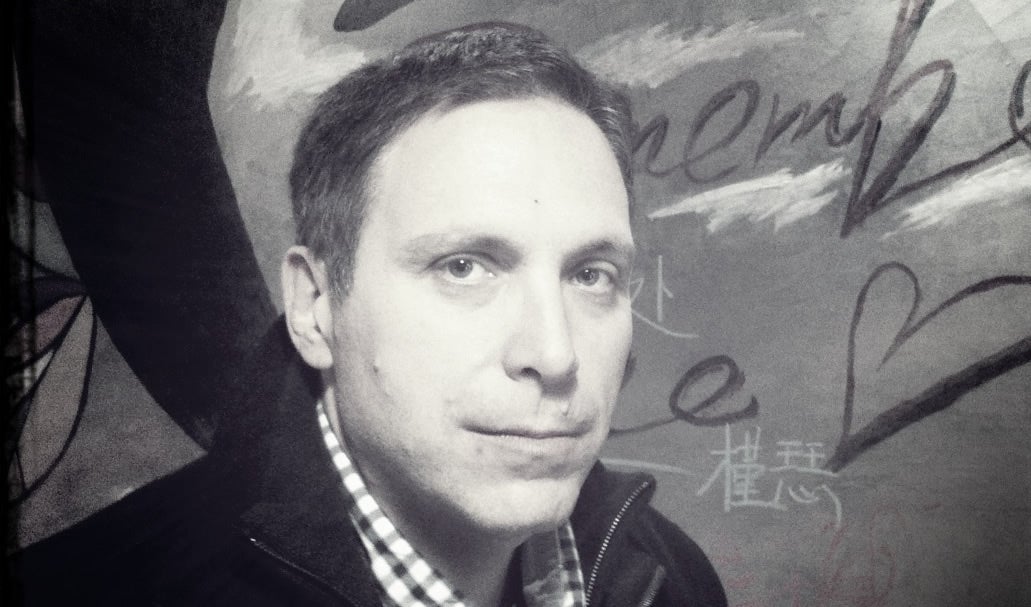
Joseph Shavit
Head Science News Writer | Communicating Innovation & Discovery
Based in Los Angeles, Joseph Shavit is an accomplished science journalist, head science news writer and co-founder at The Brighter Side of News, where he translates cutting-edge discoveries into compelling stories for a broad audience. With a strong background spanning science, business, product management, media leadership, and entrepreneurship, Joseph brings a unique perspective to science communication. His expertise allows him to uncover the intersection of technological advancements and market potential, shedding light on how groundbreaking research evolves into transformative products and industries.