Groundbreaking AI system better predicts water availability for drought-sensitive farmers
This is particularly crucial for water planners throughout the West, as “every drop of water” is allocated for various uses such as irrigation, hydropower, drinking water, etc.
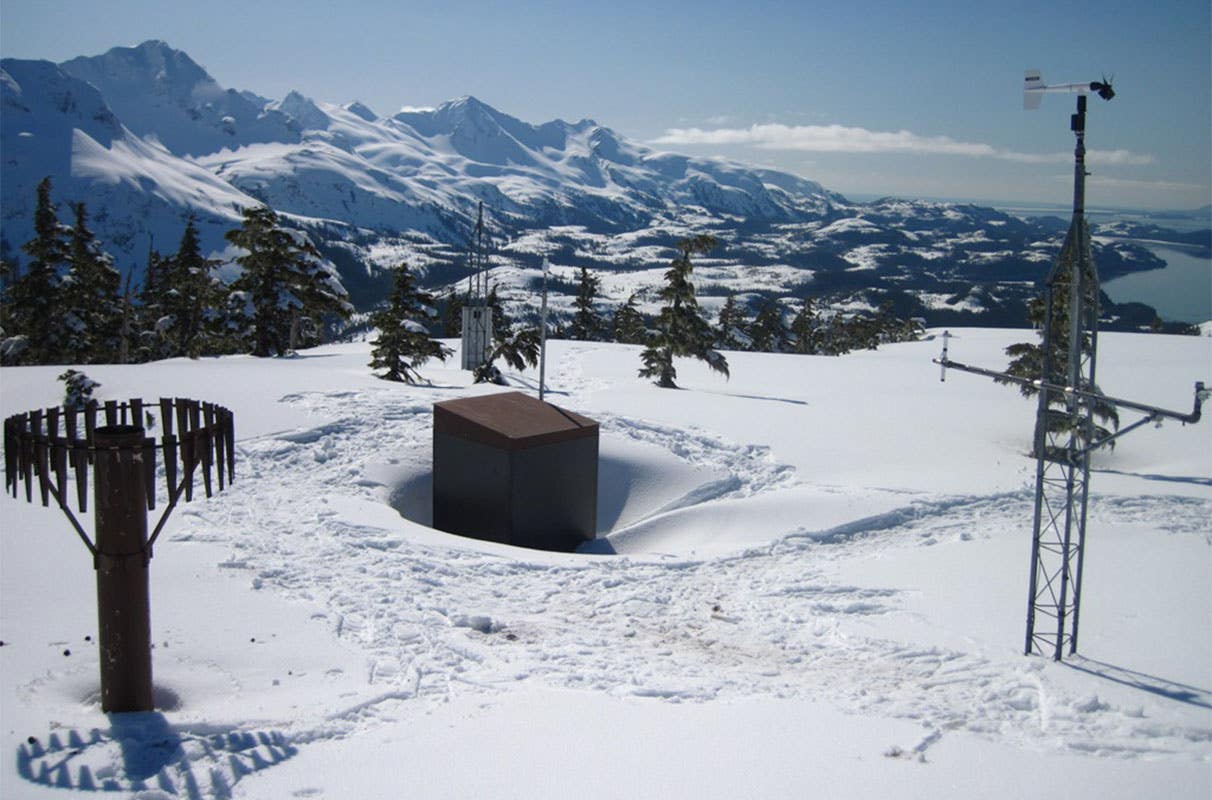
Mt. Eyak SNOTEL site, above the coastal town of Cordova, Alaska. Snow depth is about 10.5 feet, 45% density. (CREDIT: Daniel Fisher of the USDA Natural Resources Conservation Service)
A new computer model developed by an interdisciplinary team of Washington State University (WSU) researchers leverages advanced artificial intelligence (AI) to more accurately measure snow and water availability across vast distances in the Western United States. This improved model, which integrates both spatial and temporal data, holds promise for better predicting water availability for farmers and other stakeholders in the future.
Published in the Proceedings of the AAAI Conference on Artificial Intelligence, the WSU researchers' model predicts water availability in regions where physical snow measurements are not available. By comparing their results to data from over 300 snow measuring stations across the Western U.S., the team demonstrated that their model outperforms previous AI models that relied solely on machine learning.
Traditional models primarily focused on temporal data, gathering information from a few locations at different time points. In contrast, the new model incorporates both spatial and temporal data, resulting in more accurate predictions. This is particularly crucial for water planners throughout the West, as "every drop of water" is allocated for various uses such as irrigation, hydropower, drinking water, and environmental needs, according to Krishu Thapa, a WSU computer science graduate student and the study's lead author.
Each spring, water management agencies in the West make critical decisions based on the amount of snow in the mountains. "This is a problem deeply related to our way of life in the Western U.S.," said co-author Kirti Rajagopalan, a professor in WSU’s Department of Biological Systems Engineering. "Snow is key in an area where more than half of the streamflow comes from snowmelt. Understanding how snow forms, changes, and varies spatially is vital for all decisions."
There are 822 snow measurement stations throughout the Western U.S. that provide daily information on potential water availability, known as the snow-water equivalent (SWE). These stations also monitor snow depth, temperature, precipitation, and relative humidity. However, with one station approximately every 1,500 square miles, the SWE can vary significantly even a short distance from a station due to factors like topography.
"Decision makers often rely on data from nearby stations, but this doesn’t account for variations in topography and other features, leading to potential over or under predictions of water supplies," said co-author Bhupinderjeet Singh, a WSU graduate student in biological systems engineering. "Our machine learning models aim to improve these predictions."
The researchers developed a modeling framework for SWE prediction that captures spatial and temporal information to predict daily SWE for any location, regardless of station presence. Previous machine learning models focused only on temporal data, making predictions for one location over multiple days. "Using our new technique, we incorporate both spatial and temporal models to make more accurate predictions for SWE values," explained Thapa. "Our goal is to transform the sparse network of stations into dense prediction points for areas without stations."
While this model is not yet ready for direct application in decision-making, it represents a significant step toward future forecasting and improving inputs for streamflow prediction models. The researchers are working to extend the model to make it spatially complete and eventually develop it into a real-world forecasting tool.
This project was conducted through the AI Institute for Transforming Workforce and Decision Support (AgAID Institute) and supported by the USDA’s National Institute of Food and Agriculture. Rajagopalan emphasized the potential long-term benefits of the research: "As we refine the model, it will provide more accurate and comprehensive data, ultimately aiding water management and planning across the Western U.S."
By incorporating both spatial and temporal data, the model provides more accurate predictions, which are crucial for effective water management in a region where snowmelt is a key water source. The ongoing research and development aim to enhance the model’s capabilities, paving the way for more reliable and efficient water resource planning in the future.
Note: Materials provided above by the The Brighter Side of News. Content may be edited for style and length.
Like these kind of feel good stories? Get the Brighter Side of News' newsletter.
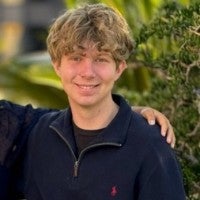
Joshua Shavit
Science & Technology Writer | AI and Robotics Reporter
Joshua Shavit is a Los Angeles-based science and technology writer with a passion for exploring the breakthroughs shaping the future. As a contributor to The Brighter Side of News, he focuses on positive and transformative advancements in AI, technology, physics, engineering, robotics and space science. Joshua is currently working towards a Bachelor of Science in Business Administration at the University of California, Berkeley. He combines his academic background with a talent for storytelling, making complex scientific discoveries engaging and accessible. His work highlights the innovators behind the ideas, bringing readers closer to the people driving progress.