Researchers revolutionize Type 1 Diabetes management with AI
People with type 1 diabetes must constantly monitor their blood glucose levels, as their bodies produce minimal or no insulin on their own.
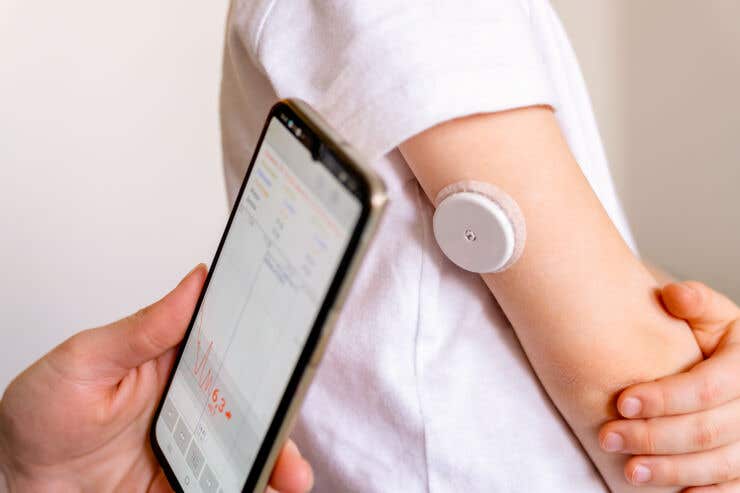
[July 7, 2023: Staff Writer, The Brighter Side of News]
People with type 1 diabetes must constantly monitor their blood glucose levels, as their bodies produce minimal or no insulin on their own. (CREDIT: Creative Commons)
Just days before his college departure, Harry Emerson faced a life-altering diagnosis – type 1 diabetes. Emerson learned that his body couldn’t produce insulin, a hormone critical for blood sugar regulation, which fuels our cells. He would require technology and medical intervention to sustain his life. Resolute to keep his college plans, Emerson hurriedly learned to navigate his new medical devices, packed his bags, and set off for university.
Emerson’s experience is not an isolated one. People with type 1 diabetes must constantly monitor their blood glucose levels, as their bodies produce minimal or no insulin on their own. They inject insulin to manage high blood sugar levels or to preempt a post-meal spike, and have quick-acting carbohydrates at hand for when blood sugar dips too low. “Every time I eat, I have to make a decision,” Emerson shares. “There are countless tiny factors that cumulatively influence my blood sugar, and it’s simply not feasible to account for them all.”
While many patients manually monitor their blood glucose levels through finger-pricks, the fortunate ones with access to advanced devices can delegate some decisions to technology. Continuous Glucose Monitors (CGMs) measure blood sugar every few minutes using a subdermal sensor, relaying readings to a handheld monitor or a smartphone.
Insulin pumps maintain steady insulin levels throughout the day, administering additional doses around mealtimes. If a CGM communicates with an insulin pump – a configuration known as a ‘closed-loop’ system – it can adjust insulin dosages to keep blood sugar within the target range, much like a thermostat controlling room temperature.
Related Stories
However, these systems aren't flawless. Relying on hard-coded control algorithms, they tend to be rigid and reactive, lacking flexibility to adjust to life’s unexpected changes. They can fail to collect data if, for instance, a patient forgets their monitor, or fails to accurately count carbohydrate intake. The impracticality of maintaining a strict routine of three meals a day is another challenge, leaving room for improvement in the technological support offered to diabetic patients.
Now pursuing his PhD at the University of Bristol’s Department of Engineering Mathematics, Emerson aims to enhance the lives of individuals with type 1 diabetes by integrating machine learning into diabetes management.
His research, published in the Journal of Biomedical Informatics, involves working with University Hospital Southampton to train machine learning algorithms, which can sustain virtual diabetes patients by making insulin dosing decisions. With a dataset comprising seven months of 30 simulated patients’ experiences, the AI managed to devise an insulin dosing strategy comparable to commercial controllers. More impressively, the AI achieved this feat using only two months of training data, significantly less than what previous algorithms needed.
Graphical Abstract: The widespread adoption of effective hybrid closed loop systems would represent an important milestone of care for people living with type 1 diabetes (T1D). (CREDIT: Biomedical Informatics)
Emerson is intrigued by machine learning’s potential to revolutionize diabetes management systems, noting that conventional control algorithms rely on lengthy patient observation and carry hefty price tags. “Current algorithms are rigid and costly to develop,” he explains, “and it might not be practical to continue in this direction.”
However, we are only at the dawn of AI-integrated diabetes management technology. Regulatory restrictions classify automated insulin delivery systems, even those devoid of AI, as high-risk medical devices in both the United States and the United Kingdom. Discussions about the regulatory requirements for emerging AI-driven systems have barely begun.
Emerson's study was a virtual experiment, as real-world testing of AI-assisted insulin delivery raises safety concerns. Assigning life-or-death insulin dosing decisions to an AI algorithm carries inherent risks. Marc Breton, a professor at the University of Virginia’s Center for Diabetes Technology who was not involved in the study, cautions, “In learning, there's always the chanceof an incorrect step being taken, which could lead to substantial differences in the output. This is both the beauty and the danger of AI.”
TD3-BC and PID performance for a varying number of training samples. The offline RL approach TD3-BC can be seen to surpass PID performance when trained on at least 5e4 samples of glucose data (100 days of glucose data). (CREDIT: Biomedical Informatics)
Emerson’s research utilized reinforcement learning (RL), a form of machine learning that relies on trial and error. In this scenario, the algorithm received 'rewards' for keeping blood glucose at target levels (good behavior) and 'punishments' for letting blood glucose get too high or low (bad behavior). The team utilized offline RL, meaning the AI learned from existing data, not by interacting in real-time.
The team synthesized 30 virtual patients (10 children, 10 adolescents, and 10 adults) using the UVA/Padova Type 1 Diabetes Simulator, an FDA-approved replacement for preclinical animal testing. After seven months of offline RL training, the AI algorithm began managing the virtual patients' insulin dosing.
To test the algorithm's robustness, they exposed it to conditions mimicking device faults (missing or incorrect data) and human errors (carb-counting mistakes, irregular meal times). “Most systems consider only a few factors: current blood glucose, previously dosed insulin, and carbohydrates,” says Emerson. The algorithm successfully navigated these challenges in the simulator, outperforming existing state-of-the-art controllers, with the most significant improvements seen in situations with missing or incorrect data.
The RL algorithm reduced training time by 90% compared to other RL algorithms and kept the virtual patients in their target blood glucose range an hour longer each day compared to commercial controllers. Emerson aims to next test the offline RL on data previously collected from actual patients, stating, “We have a considerable percentage of people with diabetes in the US and UK with their data continually recorded, providing an opportunity we can seize.”
However, there are significant barriers to overcome when transitioning from academic research to commercial application. Breton notes that while the study results look promising, they stem from virtual patients, which only represent a small fraction of our understanding of human metabolism. He underscores the significant gap between simulation studies and real-world applications, saying it's "not unbridgeable, but it’s large, and it’s necessary.”
People living with diabetes often find the development pipeline for new medical devices painfully slow. Rigorous safety testing, lack of data transparency, and incompatibility across manufacturers limit users' flexibility and choices. Access and usability are also problematic due to the high costs of the devices, with only five compatible CGM-pump pairs on the US market.
Dana Lewis, founder of the open-source artificial pancreas system movement (OpenAPS), envisions an ideal world where people can choose their pump, CGM, and controlling algorithm, allowing them to live their life without continuously thinking about their diabetes. “In an ideal world, there would be tons of systems,” says Lewis.
Community members have taken the initiative to expedite the development process. Lewis fine-tunes insulin delivery for her artificial pancreas using her historical data and shares the open-source software online to help others create their systems. “I can’t imagine managing my diabetes without it,” she admits.
Lewis doesn’t foresee RL assuming complete control of systems like hers in the immediate future. Instead, she sees machine learning supplementing existing controllers. A small fix to a tangible problem can lead to significant improvements, she argues.
One of the significant challenges in advancing AI in healthcare is demonstrating that it performs as intended. According to Daria Onitiu, a postdoctoral researcher at the Oxford Internet Institute, if a new device differs substantially from an existing one, it must undergo new certification from regulatory bodies. AI's inherent adaptability complicates this framework. Onitiu explains, “An autonomous AI algorithm can alter its internal workings and change its output.” She notes that if these alterations change the device’s intended use, itwould necessitate a re-certification.
AI already has a role in healthcare, as pointed out by Onitiu. As of October 2022, the FDA listed 521 AI-enabled medical devices available on the US market. However, these devices primarily leverage AI for tasks like analyzing urine samples or diagnosing biopsies — actions that support clinicians but don’t involve real-time medication dosing or patient treatment.
Breton's research group secured an Investigational Device Exemption from the FDA two months ago, a significant stride that will permit the testing of an AI-powered insulin pump in humans. This milestone was not easily foreseen. “Previously, it was unclear if the FDA would permit a neural net to influence insulin dosing because it’s tough to prove that it will behave as intended,” says Breton.
Despite the potential for AI to revolutionize diabetes management, Breton underscores the need for cautious progress. Academics can freely explore with fewer consequences; if a simulation fails, the implications are virtual. However, industry constraints such as safety and consumer interest must be considered. “Academia pushes the envelope, and the FDA draws boxes,” observes Breton. “But we need to be cautious when it comes to something as serious as diabetes management. There’s no room for error.”
For more science and technology stories check out our New Discoveries section at The Brighter Side of News.
Note: Materials provided above by The Brighter Side of News. Content may be edited for style and length.
Like these kind of feel good stories? Get the Brighter Side of News' newsletter.
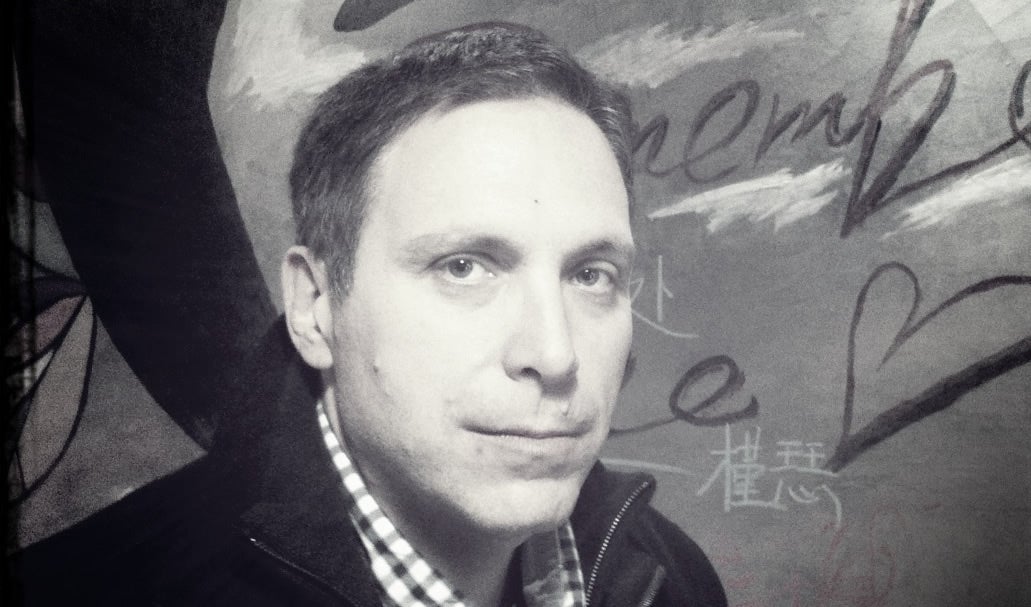
Joseph Shavit
Head Science News Writer | Communicating Innovation & Discovery
Based in Los Angeles, Joseph Shavit is an accomplished science journalist, head science news writer and co-founder at The Brighter Side of News, where he translates cutting-edge discoveries into compelling stories for a broad audience. With a strong background spanning science, business, product management, media leadership, and entrepreneurship, Joseph brings a unique perspective to science communication. His expertise allows him to uncover the intersection of technological advancements and market potential, shedding light on how groundbreaking research evolves into transformative products and industries.