Revolutionary AI discovers previously unknown antibiotics to kill lethal bacterial infections
Researchers identify a class of compounds capable of eradicating methicillin-resistant Staphylococcus aureus (MRSA) bacterium
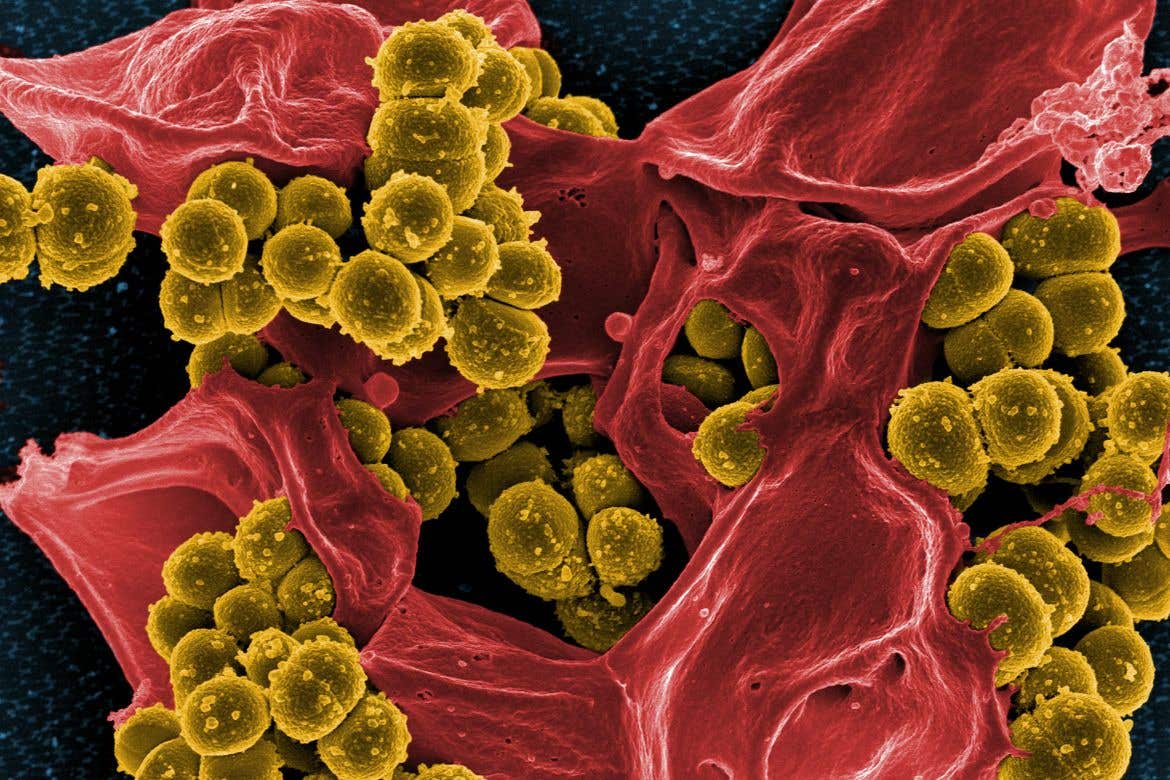
[Dec. 22, 2023: JD Shavit, The Brighter Side of News]
Researchers identify a class of compounds capable of eradicating methicillin-resistant Staphylococcus aureus (MRSA). (CREDIT: Creative Commons)
In a groundbreaking development, scientists at the Massachusetts Institute of Technology (MIT) have harnessed the power of deep learning artificial intelligence (AI) to identify a class of compounds capable of eradicating methicillin-resistant Staphylococcus aureus (MRSA), a drug-resistant bacterium responsible for more than 10,000 annual deaths in the United States alone.
Published today in the prestigious journal Nature, the research unveils a promising breakthrough in the ongoing battle against MRSA, demonstrating the compounds' efficacy in both laboratory conditions and mouse models. These compounds exhibit minimal toxicity to human cells, positioning them as strong candidates for future drug development.
A Landmark Innovation in Antibiotics Research
The heart of this remarkable study lies in its capacity to unravel the enigma of deep learning models. By peering into the "black box" of AI, MIT researchers, led by James Collins, the Termeer Professor of Medical Engineering and Science, devised a framework that unveils the underlying mechanisms guiding the AI's antibiotic potency predictions.
Related Stories
Collins explains the significance of their achievement: "The insight here was that we could see what was being learned by the models to make their predictions that certain molecules would make for good antibiotics. Our work provides a framework that is time-efficient, resource-efficient, and mechanistically insightful, from a chemical-structure standpoint, in ways that we haven't had to date."
Felix Wong, a postdoctoral researcher at MIT's Institute for Medical Engineering and Science (IMES) and the Broad Institute of MIT and Harvard, and Erica Zheng, a former Harvard Medical School graduate student, led this study as part of the Antibiotics-AI Project at MIT. The project, spearheaded by Collins, aims to discover novel antibiotic classes targeting seven lethal bacterial strains over a seven-year period.
MRSA is a formidable adversary, annually infecting over 80,000 individuals in the United States, often triggering skin infections, pneumonia, and in severe cases, sepsis—a potentially fatal bloodstream infection.
MIT, under the leadership of Collins and the Abdul Latif Jameel Clinic for Machine Learning in Health (Jameel Clinic), has increasingly incorporated deep learning into their quest for new antibiotics. Previous endeavors resulted in potential drugs against Acinetobacter baumannii and various other drug-resistant bacteria.
However, one of the main challenges faced by researchers was the inscrutability of these deep learning models, which they likened to "black boxes." These models provided predictions without any transparent insight into the factors influencing their choices. This opacity hindered scientists from understanding and potentially improving the antibiotic discovery process.
Cracking Open the Black Box
To overcome this limitation, MIT scientists embarked on a two-fold approach. First, they trained a deep learning model on an expansive dataset. This dataset included approximately 39,000 compounds tested for antibiotic activity against MRSA, along with data about the chemical structures of these compounds.
Wong elucidates: "You can represent basically any molecule as a chemical structure, and also you tell the model if that chemical structure is antibacterial or not. The model is trained on many examples like this. If you then give it any new molecule, a new arrangement of atoms and bonds, it can tell you a probability that that compound is predicted to be antibacterial."
To demystify the model's decision-making process, the researchers implemented the Monte Carlo tree search algorithm, a tool previously used to enhance explainability in deep learning models like AlphaGo. This algorithm allowed not only the prediction of each molecule's antimicrobial activity but also the identification of substructures within the molecules contributing to that activity.
Promising Antibiotic Candidates
Taking their investigation further, the team trained three additional deep learning models to predict the toxicity of compounds to various types of human cells. Combining these toxicity predictions with antimicrobial activity assessments enabled the researchers to identify compounds with potent antimicrobial properties while minimizing adverse effects on human cells.
Through this multidimensional approach, the researchers scrutinized a staggering 12 million commercially available compounds. Within this vast pool, the deep learning models pinpointed compounds from five distinct chemical classes as potential MRSA-fighting agents.
Subsequently, approximately 280 compounds were procured and tested against MRSA in a laboratory setting. This rigorous screening unveiled two compounds, belonging to the same class, as highly promising antibiotic candidates. Remarkably, these compounds reduced MRSA populations by a factor of 10 in tests conducted on two separate mouse models—one simulating MRSA skin infections and the other simulating systemic MRSA infections.
Targeting Bacterial Cell Membranes
Experiments conducted by the MIT team unveiled the mechanism by which these compounds combat MRSA. They disrupt the bacterium's ability to maintain an electrochemical gradient across their cell membranes—a crucial component of various cell functions, including ATP production, the cell's energy currency.
Interestingly, a similar antibiotic candidate, halicin, discovered by Collins' lab in 2020, operates via a comparable mechanism but targets Gram-negative bacteria with thinner cell walls. MRSA, in contrast, is a Gram-positive bacterium, characterized by thicker cell walls.
Felix Wong elaborates on the findings: "We have pretty strong evidence that this new structural class is active against Gram-positive pathogens by selectively dissipating the proton motive force in bacteria. The molecules are attacking bacterial cell membranes selectively, in a way that does not incur substantial damage in human cell membranes. Our substantially augmented deep learning approach allowed us to predict this new structural class of antibiotics and enabled the finding that it is not toxic against human cells."
The MIT researchers have shared their discoveries with Phare Bio, a nonprofit organization initiated by Collins and others as part of the Antibiotics-AI Project. Phare Bio is set to conduct in-depth analyses of the chemical properties and potential clinical applications of these compounds.
Comparison of deep learning models for predicting antibiotic 1399 activity. (CREDIT: MIT Libraries)
Meanwhile, Collins' lab remains committed to designing additional drug candidates based on the insights gleaned from this study. Additionally, they plan to leverage their deep learning models to identify compounds capable of combating other formidable bacterial foes.
Felix Wong concludes: "We are already leveraging similar approaches based on chemical substructures to design compounds de novo, and of course, we can readily adopt this approach out of the box to discover new classes of antibiotics against different pathogens."
Comparison of deep learning models for predicting human cell 1407 cytotoxicity. (CREDIT: MIT Libraries)
In a world plagued by antibiotic resistance, the marriage of artificial intelligence and innovative research holds promise in the ongoing battle against life-threatening bacterial infections.
With each breakthrough, scientists edge closer to developing novel antibiotics that can save countless lives and preserve our ability to combat infectious diseases effectively.
Note: Materials provided above by The Brighter Side of News. Content may be edited for style and length.
Like these kind of feel good stories? Get the Brighter Side of News' newsletter.
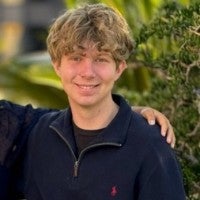
Joshua Shavit
Science & Technology Writer | AI and Robotics Reporter
Joshua Shavit is a Los Angeles-based science and technology writer with a passion for exploring the breakthroughs shaping the future. As a contributor to The Brighter Side of News, he focuses on positive and transformative advancements in AI, technology, physics, engineering, robotics and space science. Joshua is currently working towards a Bachelor of Science in Business Administration at the University of California, Berkeley. He combines his academic background with a talent for storytelling, making complex scientific discoveries engaging and accessible. His work highlights the innovators behind the ideas, bringing readers closer to the people driving progress.