Scientists create novel artificial intelligence to prevent ‘superbugs’
Scientists create (AI) model that determines the optimal combination and timing of drug prescriptions for treating bacterial infections.
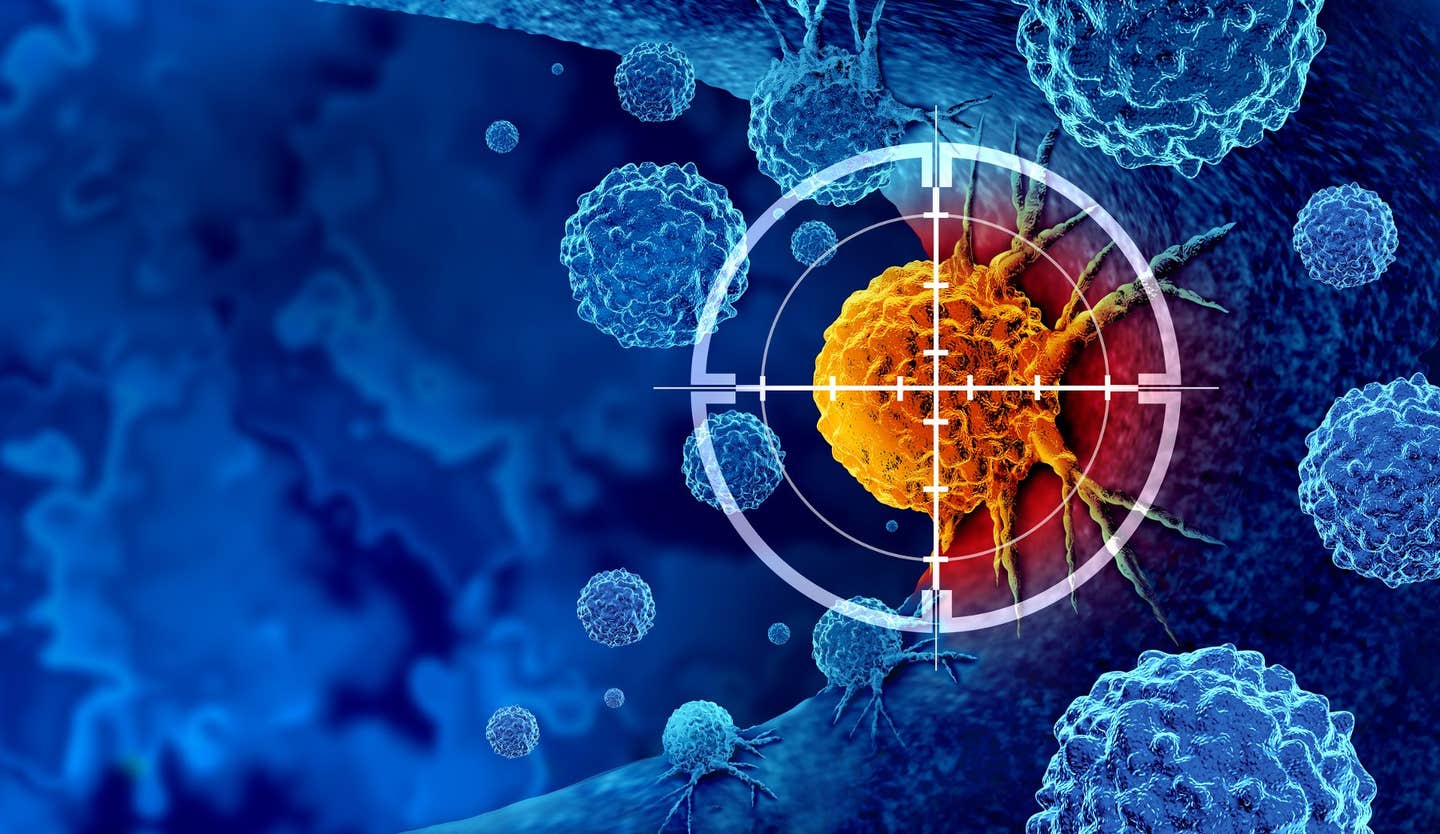
Scientists create (AI) model that determines the optimal combination and timing of drug prescriptions for treating bacterial infections. (CREDIT: Creative Commons)
Cleveland Clinic scientists have devised an artificial intelligence (AI) model that determines the optimal combination and timing of drug prescriptions for treating bacterial infections. The team, led by Dr. Jacob Scott, published their findings in the Proceedings of the National Academy of Sciences (PNAS).
Antibiotics have significantly increased the average lifespan in the US by almost a decade, reducing fatality rates for once seemingly trivial health issues. However, their effectiveness is waning due to widespread use, leading to what experts term a post-antibiotic era.
"We're entering a post-antibiotic era," warns Dr. Scott. "If we don't change how we tackle bacteria, more people will die from antibiotic-resistant infections than from cancer by 2050."
Schematic evolutionary simulation and tested optimization approaches. A simulated isogenic population of E. coli evolves on fitness landscapes in response to drug-imposed selection pressure. (CREDIT: PNAS)
Bacteria reproduce rapidly, generating mutant offspring. Excessive antibiotic usage provides opportunities for bacteria to develop mutations that resist treatment. Eventually, susceptible bacteria are eradicated, leaving behind resilient mutants.
One approach to modernizing bacterial infection treatment is antibiotic cycling, where healthcare providers alternate between different antibiotics at specific intervals. This strategy reduces the time bacteria have to evolve resistance to any particular antibiotic class and can enhance susceptibility to other antibiotics.
"Drug cycling holds promise for disease treatment," says study lead author and medical student Davis Weaver, PhD. "However, there's a lack of standardization across hospitals regarding which antibiotics to administer, for how long, and in what sequence."
Related Stories:
Dr. Jeff Maltas, a postdoctoral fellow at Cleveland Clinic, collaborates with Dr. Weaver to employ computer models predicting how resistance to one antibiotic affects susceptibility to another.
Their aim is to determine drug cycling regimens that minimize resistance and maximize susceptibility.
Dr. Weaver spearheaded the application of reinforcement learning to the drug cycling model. This technique enables a computer to learn from its successes and failures to optimize task completion. According to Drs. Weaver and Maltas, this study is among the first to apply reinforcement learning to antibiotic cycling regimens.
Performance of RL agents in a simulated E. coli system. Line plot showing the effectiveness (as measured by average population fitness) of the average learned policy as training time increases on the x-axis for RL agents trained using fitness (red) or genotype (blue). (CREDIT: PNAS)
"Reinforcement learning is ideal because it only requires knowledge of bacterial growth rates, which are relatively easy to ascertain," explains Dr. Weaver. "It accommodates human variability and errors without the need for precise growth rate measurements."
The team's AI successfully identified efficient antibiotic cycling plans for treating multiple strains of E. coli, mitigating drug resistance. Dr. Maltas highlights the potential of AI in supporting complex decision-making, such as calculating antibiotic treatment schedules.
Drug cycling policies learned by RL-genotype and RL-fit. Heatmap depicting the learned policy for 100 replicates (on the x-axis) of the RL-genotype and 100 replicates of RL-fit. (CREDIT: PNAS)
Dr. Weaver emphasizes that their AI model not only aids in managing individual infections but also informs hospital-wide infection treatment strategies. Moreover, the team is expanding their research to address other deadly diseases beyond bacterial infections.
"This concept isn't limited to bacteria; it can be extended to any evolving treatment resistance," asserts Dr. Weaver. "In the future, similar AI applications could manage drug-resistant cancers."
For more science and technology stories check out our New Innovations section at The Brighter Side of News.
Note: Materials provided above by The Brighter Side of News. Content may be edited for style and length.
Like these kind of feel good stories? Get the Brighter Side of News' newsletter.
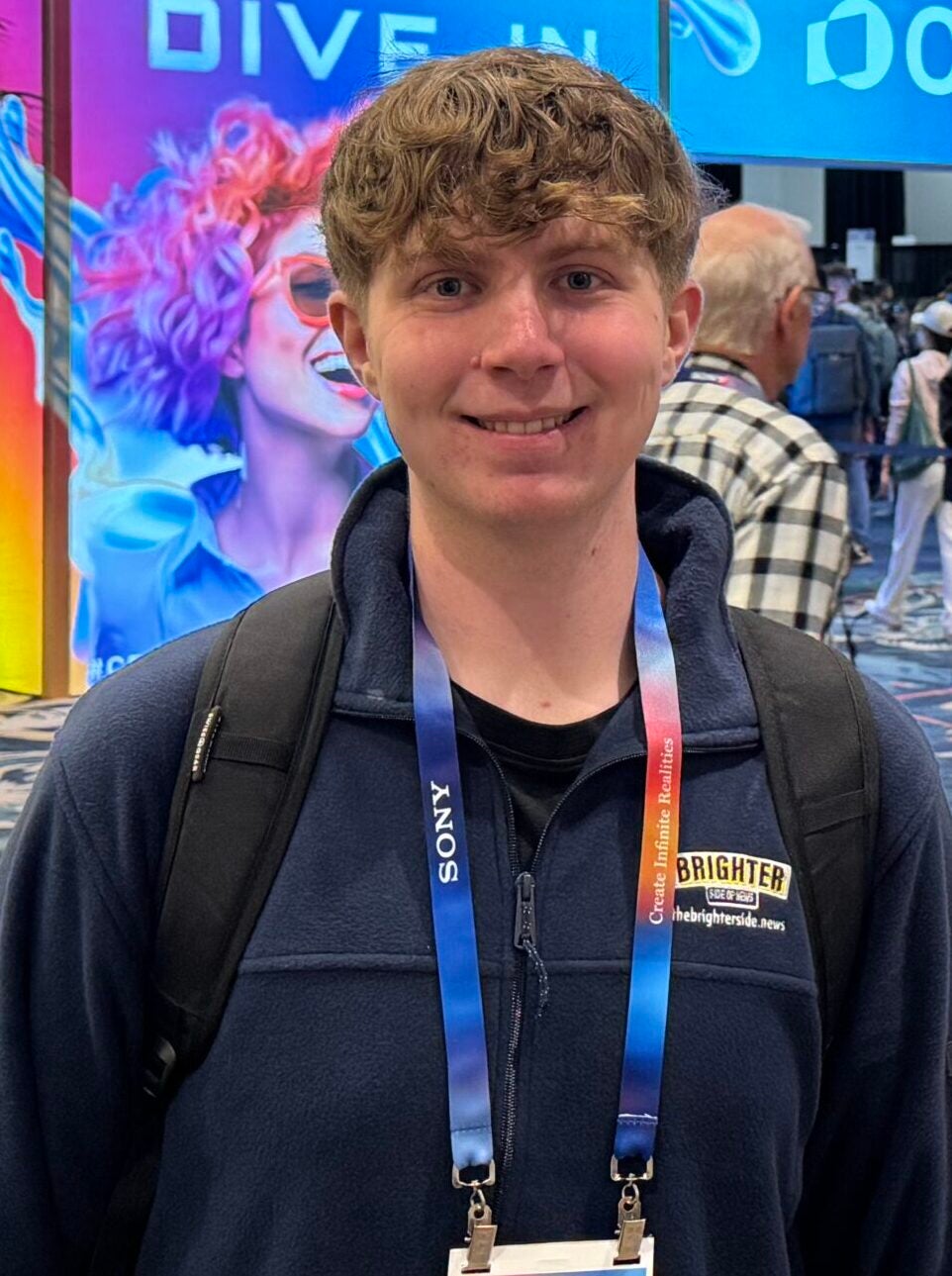
Joshua Shavit
Science & Technology Writer | AI and Robotics Reporter
Joshua Shavit is a Los Angeles-based science and technology writer with a passion for exploring the breakthroughs shaping the future. As a contributor to The Brighter Side of News, he focuses on positive and transformative advancements in AI, technology, physics, engineering, robotics and space science. Joshua is currently working towards a Bachelor of Science in Business Administration at the University of California, Berkeley. He combines his academic background with a talent for storytelling, making complex scientific discoveries engaging and accessible. His work highlights the innovators behind the ideas, bringing readers closer to the people driving progress.