What is Dark Data and what can Dark Energy teach us about it?
The more humanity tries to do more with data, the more it’s evident that the most urgent questions won’t get answered easily.
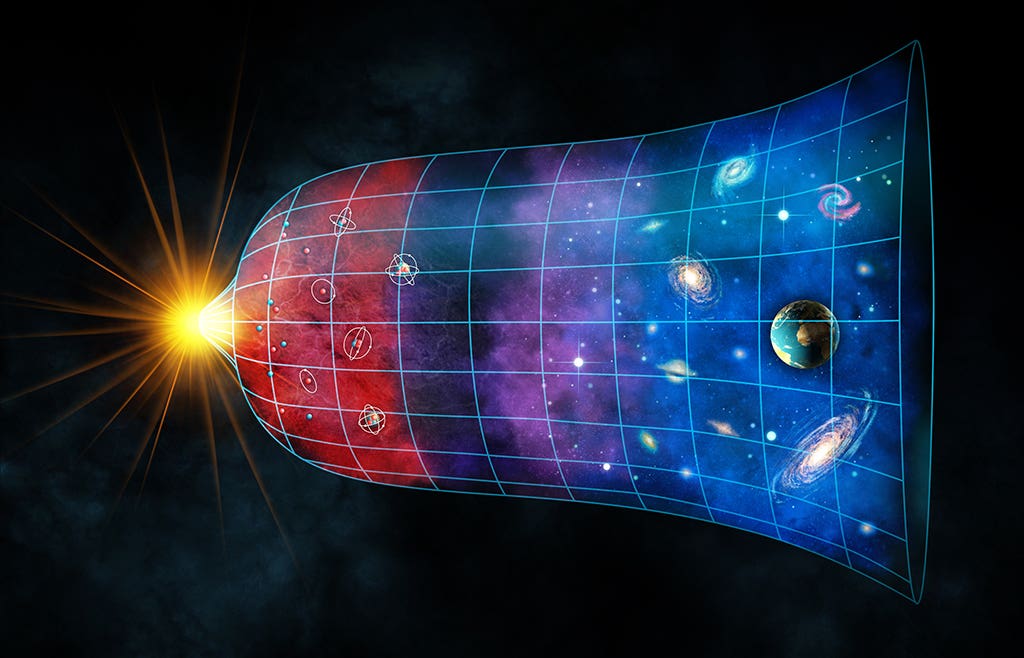
[Apr 20, 2022: Alan Morrison]
The expansion of the universe from the Big Bang to the present. Time keeps on slipping into the future. (CREDIT: Adobe Images)
During the 1990s, the physics community began to measure the brightness of certain supernovae in a novel way. This new method supported the conclusion Edwin Hubble had first arrived at in 1929 after discovering that galaxies are becoming more and more distant from us: Dark matter and dark energy play a role in why those distances are increasing. Those supernovae are growing darker.
Einstein could have drawn a similar conclusion to Hubble’s in 1917 based on some of the former’s general relativity equations. But Einstein was too cautious at that point to believe what his equations were telling him–that the universe is dynamic and expanding. According to the lambda-cold dark matter (ΛCDM) model of cosmology, dark energy is the root cause of this expansion. Advocates of this model believe that around 68 percent of the mass energy of the universe is dark energy, with 27 percent dark matter and the other five percent “normal” matter.
Scientists haven’t come to a consensus on how dark energy works yet. But a possible fifth physical force called Quintessence is one theory. The Quintessence theory asserts that the universe can either be expanding or contracting, depending on when the observations are made. Ten billion years ago, the theory went, the Quintessence force became repulsive. At other times in the past or future, that force could become attractive instead.
Another class of theories often referred to in science fiction media dwells on time warps and distortions of what scientists believe they’re computing and perceiving.
Related Stories
It’s good to be skeptical about perception. But I side with those who conclude that dark energy is still in repulsive mode. In other words, Hubble’s 1929 observations more or less accurately uncovered the reality. The universe is expanding at an accelerating rate…and growing darker.
According to the lambda-cold dark matter (ΛCDM) model of cosmology, dark energy is the root cause of this expansion. (CREDIT: Wikimedia Commons, 2012)
The dark energy analogy for “dark” data
In 1997, Dame Frances Cairncross published a book called The Death of Distance that explored the impact of the communications revolution. Of course, the world has seemed smaller because of the growth of the web and other advances related to better computing, networking, and storage.
But the web and intelligence haven’t gone nearly as far as they need to. They’ll need to start answering life’s most persistent and urgent questions and provide ways to find better questions to ask.
The more humanity tries to do more with data, the more it’s evident that the most urgent questions that demand some form of data retrieval, integration, and analysis won’t get answered easily. And it’s not just a matter of having the answers–it’s the challenge of providing enough clues to those who need specific answers so that they’re not just hunting around in the dark.
In quite a few cases, the right data (or at least some helpful data) does exist, but getting the right meaning out of it and delivering that meaning to the right people in a helpful way and in a timely fashion just isn’t possible.
I call this data “dark” because it’s data that could be illuminating in more contexts but isn’t. It’s disconnected, underexplored or underutilized data. It lacks precision, and it’s not sufficiently contextualized. (These last two phenomena are related, BTW–if you lack logically constructed context, by definition, you lack precision.)
Dark data, in short, doesn’t shed the light it needs on problems or solutions.
Reducing data distance to add illumination and yield more value
Data distance in this post means the distance between key data consumers and the data they need most to address the problems they’re responsible for. Some of the wrong assumptions enterprises frequently make that prevent them from reducing data distance include these:
The fatal mistake enterprises tend to make repeatedly is diminishing the value of different forms of heterogeneous data brought together. Enterprises should meld the less structured with, the more structured and the external with the internal. Semantic graphs allow heterogeneous data integration at scale because semantic graphs can serve as the parents of the other data model children.
Reducing data distance has already proven to be powerful. Diffbot crawls the entire web to yield eCommerce insights for its customers. Montefiore Health has expanded, articulated, and mined its knowledge graph for different new varieties of personalized or patient cohort-specific insights every year since 2016.
In this way, holistic, global knowledge + data management techniques have been feasible at an enterprise scale for years now. Unfortunately, few in data management seem to realize or acknowledge that knowledge, content, and data management is possible using one type of standardized, mature knowledge graph technique. Three departments are no longer necessary–one suffices. Unifying management in this way can clear a path to sharable data that sheds more light, regardless of the end-user.
For more science stories check out our New Discoveries section at The Brighter Side of News.
Note: Materials provided above by Alan Morrison. Content may be edited for style and length.
Like these kind of feel good stories? Get the Brighter Side of News' newsletter.
Tags: #New_Discoveries, #Universe, #Astrophysics, #Cosmology, #Galaxies, #Dark_Energy, #Dark_Data, #Science, #Research, #The_Brighter_Side_of_News
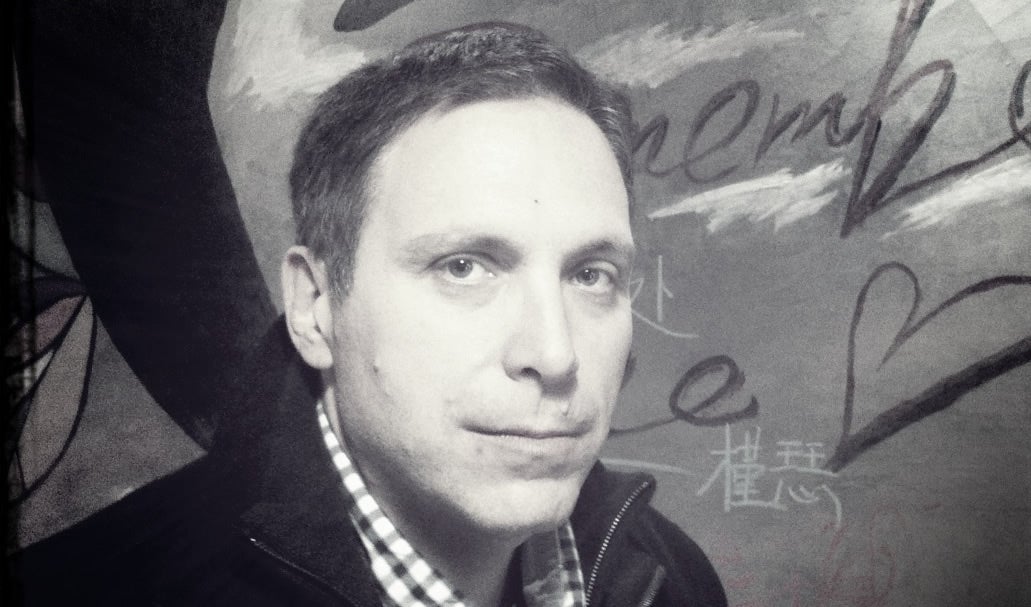
Joseph Shavit
Head Science News Writer | Communicating Innovation & Discovery
Based in Los Angeles, Joseph Shavit is an accomplished science journalist, head science news writer and co-founder at The Brighter Side of News, where he translates cutting-edge discoveries into compelling stories for a broad audience. With a strong background spanning science, business, product management, media leadership, and entrepreneurship, Joseph brings a unique perspective to science communication. His expertise allows him to uncover the intersection of technological advancements and market potential, shedding light on how groundbreaking research evolves into transformative products and industries.