YouTube’s algorithm isn’t radicalizing viewers, new study concludes
YouTube, one of the largest online media platforms globally, draws about a quarter of Americans for news consumption.
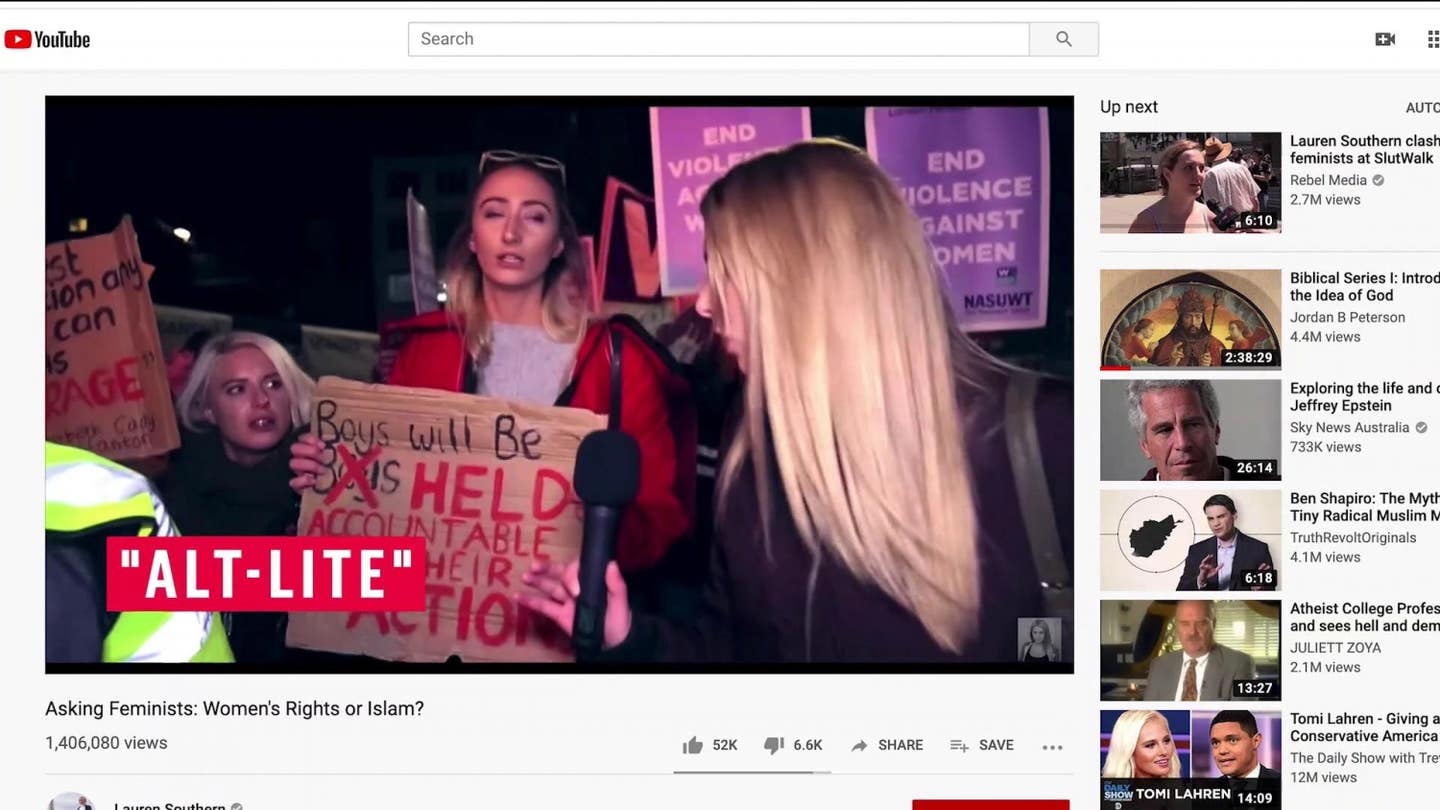
YouTube, one of the largest online media platforms globally, draws about a quarter of Americans for news consumption.
Concerns have surfaced in recent years regarding the potential radicalization of young Americans by highly partisan and conspiracy-driven channels on YouTube, with critics blaming the platform's recommendation algorithm for leading users towards increasingly extreme content.
However, a new study from the Annenberg School for Communication's Computational Social Science Lab (CSSLab) challenges this narrative, suggesting that users' own political interests primarily shape their viewing habits, with the recommendation feature potentially moderating their consumption.
Lead author Homa Hosseinmardi, an associate research scientist at CSSLab, highlights that reliance solely on YouTube's recommendations tends to result in less partisan consumption on average.
Related Stories
To investigate the impact of YouTube's recommendation algorithm, researchers developed bots that either followed or disregarded recommendations, using the watch history of nearly 88,000 real users collected from October 2021 to December 2022.
The study, led by Hosseinmardi and co-authors including Amir Ghasemian, Miguel Rivera-Lanas, Manoel Horta Ribeiro, Robert West, and Duncan J. Watts, aimed to unravel the intricate relationship between user preferences and the recommendation algorithm, which evolves with each video watched.
These bots were assigned individualized YouTube accounts to track their viewing history, with the partisanship of their content estimated using video metadata.
In two experiments, the bots underwent a learning phase where they watched a standardized sequence of videos to present uniform preferences to the algorithm. Subsequently, they were divided into groups: some continued to follow real users' behavior, while others acted as experimental "counterfactual bots," adhering to specific rules to isolate user behavior from algorithmic influence.
In the first experiment, counterfactual bots deviated from real users' behavior by solely selecting videos from recommendations, without considering user preferences. The researchers observed that these bots consumed less partisan content on average compared to corresponding real users, indicating an intrinsic user preference for such content relative to algorithmic recommendations.
Additionally, experiment two aimed to estimate the "forgetting time" of the YouTube recommender, addressing concerns about the algorithm's persistence in recommending content even after users lose interest.
Researchers found that recommendations on the sidebar shifted towards moderate content after approximately 30 videos, while homepage recommendations adjusted less rapidly, suggesting a preference alignment with the video being watched.
Hosseinmardi acknowledges the accusations against YouTube's recommendation algorithm for promoting conspiratorial beliefs but emphasizes users' agency in their actions. He suggests that users might have viewed similar content, with or without recommendations.
Looking ahead, the researchers advocate for the adoption of their methodology to study AI-mediated platforms, aiming to better understand the role of algorithmic content recommendation engines in daily life.
For more science news stories check out our New Discoveries section at The Brighter Side of News.
Note: Materials provided by The Brighter Side of News. Content may be edited for style and length.
Like these kind of feel good stories? Get the Brighter Side of News' newsletter.
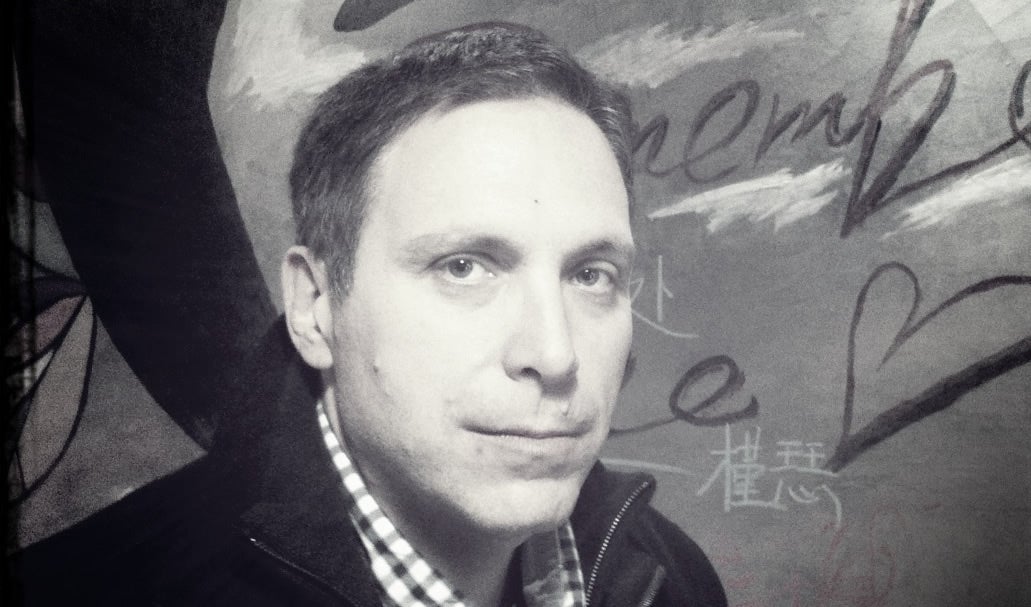
Joseph Shavit
Head Science News Writer | Communicating Innovation & Discovery
Based in Los Angeles, Joseph Shavit is an accomplished science journalist, head science news writer and co-founder at The Brighter Side of News, where he translates cutting-edge discoveries into compelling stories for a broad audience. With a strong background spanning science, business, product management, media leadership, and entrepreneurship, Joseph brings a unique perspective to science communication. His expertise allows him to uncover the intersection of technological advancements and market potential, shedding light on how groundbreaking research evolves into transformative products and industries.