Major discovery cuts the carbon footprint of AI training by up to 75%
Deep learning models that power giants like TikTok and Amazon, as well as tools like ChatGPT, could save energy without new hardware.
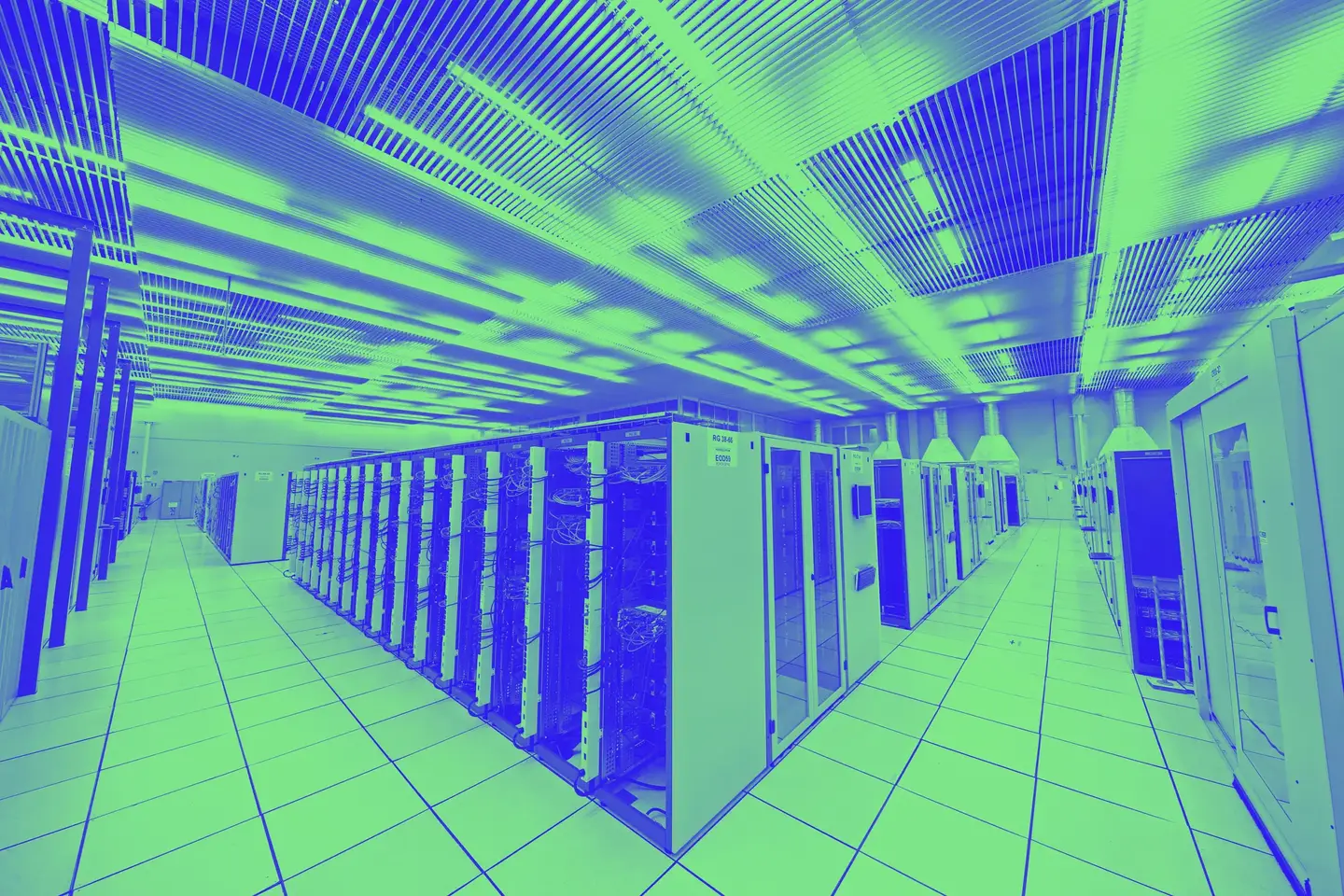
[Apr. 23, 2023: JD Shavit, The Brighter Side of News]
Deep learning models that power giants like TikTok and Amazon, as well as tools like ChatGPT, could save energy without new hardware. (CREDIT: Creative Commons)
Artificial intelligence (AI) has been a rapidly evolving tool in recent years, with deep learning models playing a crucial role in powering AI. However, the energy demands of these models are immense, with some models consuming as much energy as an average US household uses in 120 years.
To combat this issue, researchers at the University of Michigan have developed an open-source optimization framework called Zeus, which studies deep learning models during training to find the best tradeoff between energy consumption and the speed of training.
The Zeus framework was presented at the 2023 USENIX Symposium on Networked Systems Design and Implementation (NSDI) in Boston. It has the potential to reduce the energy consumption of deep learning models by up to 75% without requiring any new hardware and with only minor impacts on training time.
Mainstream uses for hefty deep learning models have exploded over the past three years, ranging from image-generation models and expressive chatbots to the recommender systems powering TikTok and Amazon. With cloud computing already out-emitting commercial aviation, the increased climate burden from artificial intelligence is a significant concern.
Related Stories
Jae-Won Chung, a doctoral student in computer science and engineering and co-first author of the study, stated that "existing work primarily focuses on optimizing deep learning training for faster completion, often without considering the impact on energy efficiency. We discovered that the energy we're pouring into GPUs is giving diminishing returns, which allows us to reduce energy consumption significantly, with relatively little slowdown."
Deep learning is a family of techniques making use of multilayered, artificial neural networks to tackle a range of common machine learning tasks. These are also known as deep neural networks (DNNs).
The models themselves are extremely complex, learning from some of the most massive data sets ever used in machine learning. Because of this, they benefit greatly from the multitasking capabilities of graphical processing units (GPUs), which burn through 70% of the power that goes into training one of these models.
Zeus uses two software knobs to reduce energy consumption. One is the GPU power limit, which lowers a GPU's power use while slowing down the model's training until the setting is adjusted again. The other is the deep learning model's batch size parameter, which controls how many samples from the training data the model works through before updating the way the model represents the relationships it finds in the data. Higher batch sizes reduce training time, but with increased energy consumption. Zeus is able to tune each of these settings in real time, seeking the optimal tradeoff point at which energy usage is minimized with as little impact on training time as possible.
In examples, the team was able to visually demonstrate this tradeoff point by showing every possible combination of these two parameters. While that level of thoroughness won't happen in practice with a particular training job, Zeus will take advantage of the repetitive nature of machine learning to come very close. "Fortunately, companies train the same DNN over and over again on newer data, as often as every hour. We can learn about how the DNN behaves by observing across those recurrences," said Jie You, a recent doctoral graduate in computer science and engineering and co-lead author of the study.
A variety of common deep learning models benefit from Zeus’ ability to tune GPU power limits and the training batch size. When both parameters were tuned, the software achieved up to 75% energy reduction. (CREDIT: SymbioticLab, University of Michigan)
Zeus is the first framework designed to plug into existing workflows for a variety of machine learning tasks and GPUs, reducing energy consumption without requiring any changes to a system's hardware or data center infrastructure.
The team has also developed complementary software called Chase, which can further reduce the carbon footprint. Chase prioritizes speed when low-carbon energy is available and chooses efficiency at the expense of speed during peak times when carbon-intensive energy generation such as coal is more likely to be used. Chase took second place at last year's CarbonHack hackathon and is now set to be presented at the International Conference on Learning Representations Workshop.
The combination of Zeus and Chase offers a promising solution to reducing the carbon footprint of deep learning models. By optimizing the energy consumption during training and selecting the most environmentally friendly energy source, the research team hopes to address the concerns over the increasing climate burden of AI.
The team has already tested Zeus and Chase on several models, including the widely used ResNet-50 image recognition model. The results showed that the energy consumption could be reduced by up to 75% without significantly affecting the model's training time or accuracy. Moreover, by using Chase, the team was able to reduce the carbon footprint of the model by up to 95%.
The potential impact of this research is significant. As the use of deep learning models becomes increasingly prevalent in various industries, the demand for energy to train these models is expected to grow exponentially. A recent study estimated that the energy consumption of AI could increase by up to 300% by 2025, leading to a substantial increase in greenhouse gas emissions.
However, with the development of energy-efficient and environmentally conscious optimization frameworks such as Zeus and Chase, there is hope that the impact of AI on the environment can be mitigated. The open-source nature of the frameworks means that they are accessible to researchers and practitioners worldwide, allowing for widespread adoption and further development.
The team at the University of Michigan hopes that their research will inspire others to prioritize energy efficiency and sustainability in AI development. "We believe that researchers and practitioners in the AI community have a responsibility to ensure that our field does not contribute to the climate crisis," said Chowdhury. "We hope that our work can serve as a model for others to follow."
For more good news stories check out our Good News section at The Brighter Side of News.
Note: Materials provided above by The Brighter Side of News. Content may be edited for style and length.
Like these kind of feel good stories? Get the Brighter Side of News' newsletter.
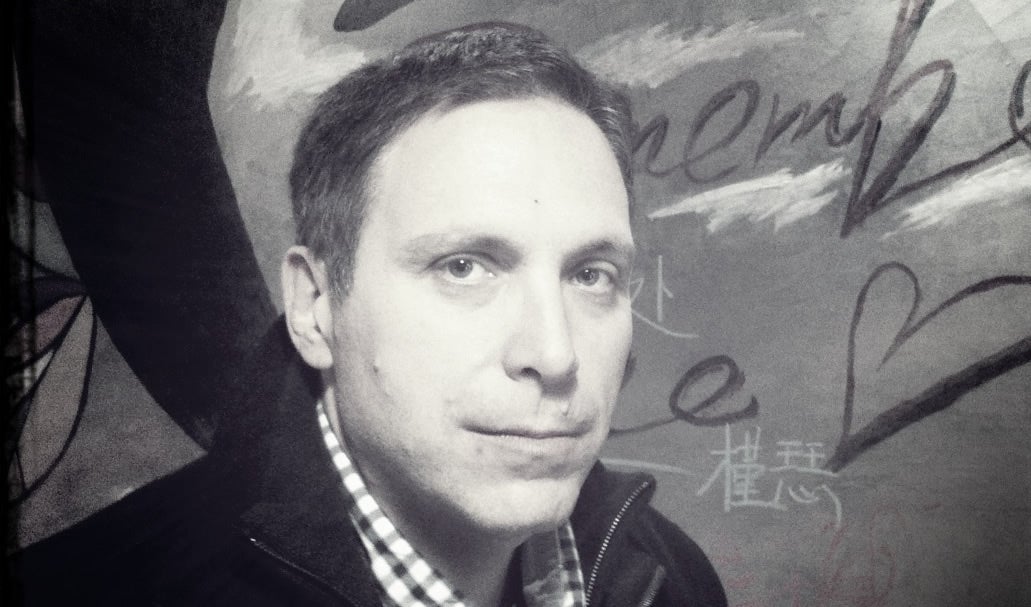