Groundbreaking AI technology could improve early breast cancer detection by 30%
Artificial intelligence could help detect these cancers sooner—sometimes even before they become visible to the human eye.
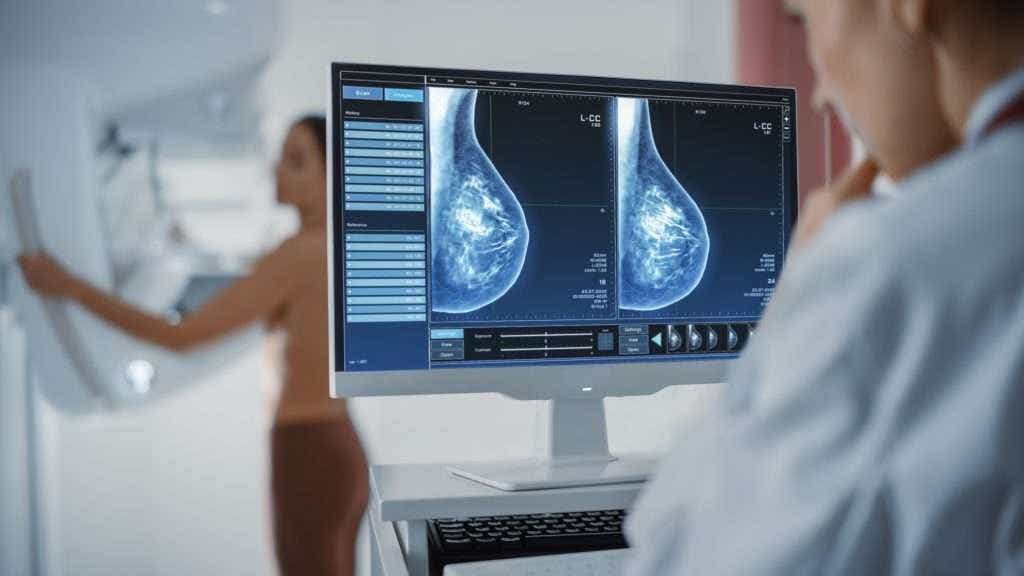
AI could detect interval breast cancers missed by radiologists. (CREDIT: Shutterstock)
In the space between scheduled mammograms, some breast cancers can silently grow and spread. These are known as interval breast cancers, or IBCs. Unlike cancers caught during a regular screening, interval cancers are usually discovered because a patient begins experiencing symptoms before her next mammogram. These cancers tend to be more aggressive and harder to treat, which means timing is everything.
A recent study from the UCLA Health Jonsson Comprehensive Cancer Center suggests that artificial intelligence could help detect these cancers sooner—sometimes even before they become visible to the human eye. With the help of AI, researchers believe it's possible to reduce the number of missed diagnoses and improve outcomes for many patients.
AI Offers a Second Look at Missed Signs
Dr. Tiffany Yu, assistant professor of Radiology at UCLA and lead author of the study, explains what makes IBCs so challenging: “These interval cancer types could be caught earlier when the cancer is easier to treat. For patients, catching cancer early can make all the difference. It can lead to less aggressive treatment and improve the chances of a better outcome.”
Interval cancers can develop between yearly screenings in the U.S., where patients typically undergo digital breast tomosynthesis, also known as 3D mammography. In contrast, most European countries rely on digital mammography, or 2D screening, and recommend screening every two or three years. The differences in timing and technology can affect how and when these cancers are caught.
Unlike many European programs, U.S. screening programs do not routinely classify interval cancers. This gap has made it difficult to assess how well screening methods are working or how tools like AI could help. The UCLA study aimed to address that problem.
A Look at the Data
The research team reviewed nearly 185,000 mammograms from 2010 to 2019. From these, they identified 148 cases of interval breast cancer. Using a classification system borrowed from European guidelines, radiologists then categorized the cancers based on how they appeared—or didn’t—on earlier scans.
Related Stories
These categories included:
- Missed Reading Error: The cancer was visible but overlooked.
- Minimal Signs (Actionable and Non-Actionable): The cancer showed faint signs that could have been detected or were likely too subtle to notice.
- Occult: The cancer was not visible on the mammogram but might be detectable using other imaging methods.
- True Interval Cancer: The cancer truly wasn’t present at the time of the last screening but developed quickly afterward.
- Technical Error: The scan itself was flawed.
Researchers then applied an AI tool, called Transpara, to these earlier mammograms. The AI scored each scan on a scale of 1 to 10 for cancer risk. Anything above an 8 was flagged as suspicious.
The results were striking. The AI tool flagged 76% of the mammograms that had originally been read as normal but were later linked to an interval cancer. That includes:
- 90% of the missed reading error cases.
- 89% of the minimal-signs-actionable cancers.
- 72% of the minimal-signs-non-actionable cancers.
- 69% of the occult cancers.
- 50% of the true interval cancers.
In other words, the AI often caught something that the radiologist didn’t—especially when the signs were subtle.
Where the AI Struggles
Still, the tool had its limits. Dr. Hannah Milch, senior author of the study and also from UCLA, pointed out that while the AI flagged many occult cancers, it struggled to pinpoint exactly where the cancer was.
“For example, despite being invisible on mammography, the AI tool still flagged 69% of the screening mammograms that had occult cancers,” she said. “However, when we looked at the specific areas on the images that the AI marked as suspicious, the AI did not do as good of a job and only marked the actual cancer 22% of the time.”
This discrepancy shows that while the AI tool may be helpful in alerting doctors to potential risks, it shouldn’t be used as a standalone decision-maker. Instead, it could act as a second set of eyes to help radiologists focus their attention, especially in cases where abnormalities are easy to miss.
Dr. Yu agrees. “While AI isn’t perfect and shouldn’t be used on its own, these findings support the idea that AI could help shift interval breast cancers toward mostly true interval cancers,” she said. That would mean more cancers caught at an earlier stage and fewer that go undetected until symptoms arise.
The Bigger Picture
Interval breast cancers are not rare. Depending on the program and technology used, they can make up anywhere from 10% to 38% of all cancers detected in screened populations. In some reports, IBC rates range from 7 to nearly 50 per 10,000 screens.
Even more confusing is the definition of a “false negative” mammogram—a scan that looks normal at first but shows clear signs of cancer in hindsight. Some studies treat false negatives as a type of IBC; others list them separately. As a result, reported false negative rates swing wildly from 4% to 40%.
Adding to the confusion is the wide variety in how screening programs are run. Factors like screening intervals, imaging technology, the number of readers, and even radiologist training can all influence outcomes. That’s why standardizing how interval cancers are reviewed and classified is so important.
UCLA’s study is one of the first in the U.S. to apply a formal classification method to a large screening cohort and test how AI performs on real cases. While previous research has come mainly from European systems, this work shows how AI might function in an American context, where annual screening and 3D imaging are more common.
Next Steps for AI in Breast Cancer Screening
The findings offer a hopeful glimpse into what AI might offer radiologists and patients alike. Used alongside trained professionals, AI tools could help flag subtle signs of cancer and give doctors a chance to act before a tumor becomes harder to treat. But more research is needed to refine how these tools are used and to answer big questions about their accuracy and reliability in clinical settings.interval breast cancer detection AI
“There’s a lot we still don’t know,” said Dr. Milch. “Larger prospective studies are needed to understand how radiologists would use AI in practice and how to handle cases where AI flags areas as suspicious that aren’t visible to the human eye.”
Researchers from UCLA who contributed to the study include Dr. Anne Hoyt, Dr. Melissa Joines, Dr. Cheryce Fischer, Dr. Nazanin Yaghmai, Dr. James Chalfant, Dr. Lucy Chow, Dr. Shabnam Mortazavi, Christopher Sears, Dr. James Sayre, Dr. Joann Elmore and Dr. William Hsu. The study was supported by the National Institutes of Health, the National Cancer Institute, the Agency for Healthcare Research and Quality, and Early Diagnostics Inc.
As more tools like this become available, the hope is clear: fewer missed cancers, earlier treatments, and better outcomes for patients.
Note: The article above provided above by The Brighter Side of News.
Like these kind of feel good stories? Get The Brighter Side of News' newsletter.
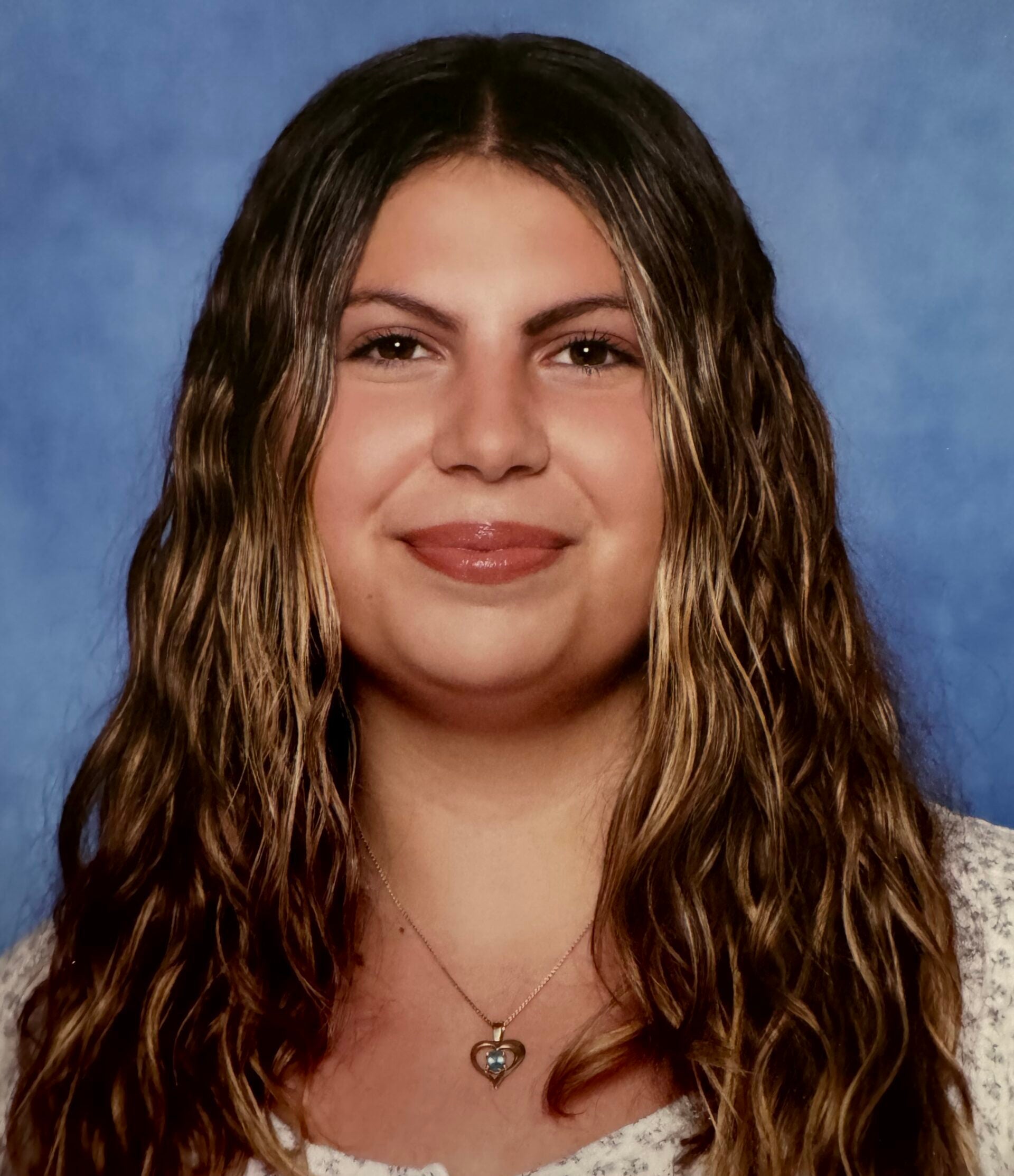
Rebecca Shavit
Science & Technology Journalist | Innovation Storyteller
Based in Los Angeles, Rebecca Shavit is a dedicated science and technology journalist who writes for The Brighter Side of News, an online publication committed to highlighting positive and transformative stories from around the world. With a passion for uncovering groundbreaking discoveries and innovations, she brings to light the scientific advancements shaping a better future. Her reporting spans a wide range of topics, from cutting-edge medical breakthroughs and artificial intelligence to green technology and space exploration. With a keen ability to translate complex concepts into engaging and accessible stories, she makes science and innovation relatable to a broad audience.