New AI predicts how enzymes behave – eliminates the need for live cell experiments
A new method combines machine learning and cell-free systems to design powerful enzymes faster than ever—no living cells needed.
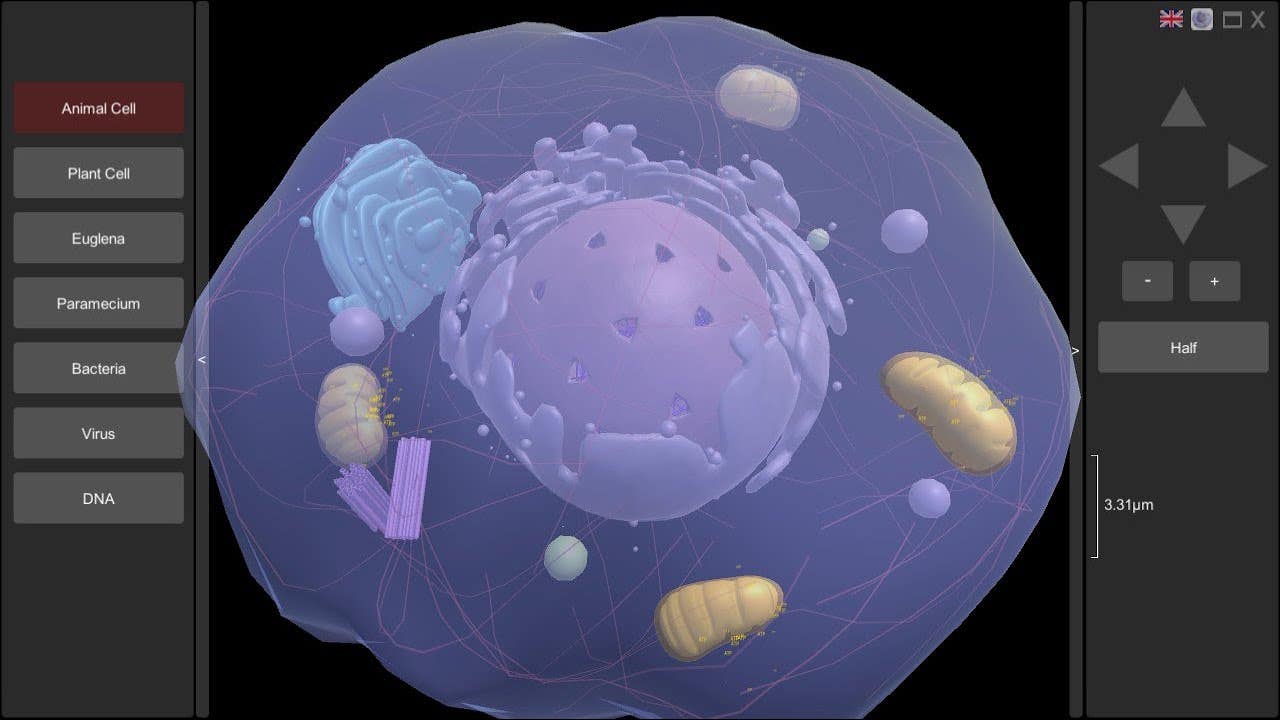
Machine learning speeds enzyme design by skipping cells, cutting testing time from months to days. (CREDIT: CC BY-SA 4.0)
Enzymes help digest food, clean laundry, make perfumes, and build medicines. They also break down toxins in the environment. Because of their wide use, scientists have worked for years to create better, faster, and more useful enzymes. But the process has always been slow and difficult—until now.
Scientists at Stanford University have created a faster way to design enzymes. They use machine learning, computer models, and “cell-free systems” to predict how enzymes will behave. This lets them test thousands of designs on a computer before building the best ones in a lab.
“We’ve developed a computational process that allows us to engineer enzymes much faster, because we don’t have to use living cells,” said Michael Jewett, a bioengineering professor and lead author of the study. “Instead, we use machine learning to predict highly active designer enzymes that have been engineered from mutated DNA sequences modeled on the computer instead of created by hand in the lab. We can carry out these experiments in days rather than weeks or, as is often the case, months.”
Their new method, published in Nature Communications, could reshape enzyme science. By skipping the use of live cells and relying on computers, the team speeds up a process that once took months—now down to just a few days.
Building Enzymes Without Cells
The traditional method of making enzymes starts with something nature already created. Scientists then tweak its DNA again and again to try to improve it. They send out for new DNA, insert it into cells, and then grow those cells to produce enzymes. After that, they must extract and purify each enzyme before testing it. This can take months—and often doesn't lead to success.
Now, things are different. Jewett's team skips the cells entirely. They use "cell-free systems," which are mixtures that can read DNA and produce proteins without needing a whole living organism. These systems are faster and easier to control.
Using this setup, the scientists test thousands of enzyme designs in many different chemical reactions. In this study, they focused on a group of enzymes called amide synthetases. These enzymes form bonds called amide bonds, which are found in many medicines and food molecules.
Related Stories
By evaluating 1,217 different versions of these enzymes in 10,953 reactions, the team gathered a large amount of data. This helped their machine learning models get better at predicting which enzyme designs would work best.
The models used a method called "augmented ridge regression" to predict which changes in DNA would lead to better enzyme activity. Then, they created enzymes designed to make nine small-molecule drugs. The results were striking. In some cases, the new enzymes worked 42 times better than the original ones.
Speeding Up Evolution
The field that Jewett and others work in is called "directed evolution." It mimics the way natural evolution works, only much faster. Instead of waiting for random mutations over millions of years, scientists introduce changes to DNA in the lab, hoping to improve the resulting proteins.
“Directed evolution is a decades-old field that has developed the ability to mutate amino acids to change the function of the protein. We’re just speeding up the process using machine learning and computers,” Jewett explained.
Enzymes are proteins made from strings of amino acids. These strings fold into shapes that determine how they work. The sequence of amino acids comes from DNA. Change the DNA, and the enzyme can behave differently. But predicting which changes will help is hard. That's where machine learning steps in.
Instead of testing one change at a time, computers can model many changes at once and predict the results. That way, scientists can skip most of the trial-and-error work. According to Jewett, “Rather than having to run 10,000 chemical reactions to iteratively improve enzyme activity, we can use machine learning models to predict highly active variants that still do just as well.”
Challenges Remain
The system isn’t perfect. One big challenge is the lack of data. Machine learning depends on huge amounts of data to train its models. But in enzyme research, that data is often hard to find.
“In the context of directed evolution and biocatalysis, generating large amounts of data for carrying out those chemical reactions is not something that is commonly reported in the scientific literature,” Jewett said. “The process of generating the data is just too slow.”
Like these kind of feel good stories? Get The Brighter Side of News' newsletter.
In this study, the team managed to test about 3,000 enzyme mutants across around 10,000 chemical reactions. But even that is not enough. Jewett pointed out that many studies only report data for a few enzyme changes, maybe ten. To reach full potential, researchers need data on tens of thousands of variants.
“If I wanted to mutate an enzyme to test tens of thousands of variants,” he said, “I might find papers out there, but they may report mutant data for ten variants. Not hundreds. Not thousands. Not tens of thousands of reactions, but ten. So, we have a way to go on the data front, but we’ll get there. This is the first step.”
New Possibilities
Despite the data hurdle, the platform offers major promise. As a test case, the team used their method to make a small-molecule drug with 90% yield. This was up from an earlier yield of just 10%. Even more exciting, they showed the same approach can be used to make eight other drugs, all using specially designed enzymes.
That’s just the beginning. Jewett sees many future uses. One goal is to design enzymes that can break down toxins in the environment. Others might help make protein-rich foods easier to digest. Some could replace chemical processes that now require high pressure, expensive parts, or toxic substances—making them safer and cheaper.
“We could explore multiple opportunities in sustainability and the bioeconomy,” Jewett said. “You could begin thinking about classes of molecules that degrade toxins from the environment, enhance availability of protein-rich foods, or others that take existing processes that require high pressures, costly components, or toxic reactions and make them faster, safer, and less expensive.”
Jewett’s group is now looking for partners in the drug industry to help test the platform further. They also want to expand the system to work with more types of chemical reactions beyond amide bond formation.
What’s Next?
This research marks an important step forward in how we create enzymes. With faster testing and better predictions, scientists can now dream bigger. Enzymes that fight disease, clean the planet, and make products more efficiently are closer than ever.
By combining machine learning with cell-free systems, scientists have created a new path for protein engineering. One that’s quicker, more flexible, and ready to scale.
As the field moves forward, it will need more data, more computer power, and more collaboration. But the potential is enormous. And it all starts with understanding that enzymes—tiny molecules in our bodies and in nature—might just hold the key to solving some of our biggest problems.
Note: The article above provided above by The Brighter Side of News.
Like these kind of feel good stories? Get The Brighter Side of News' newsletter.
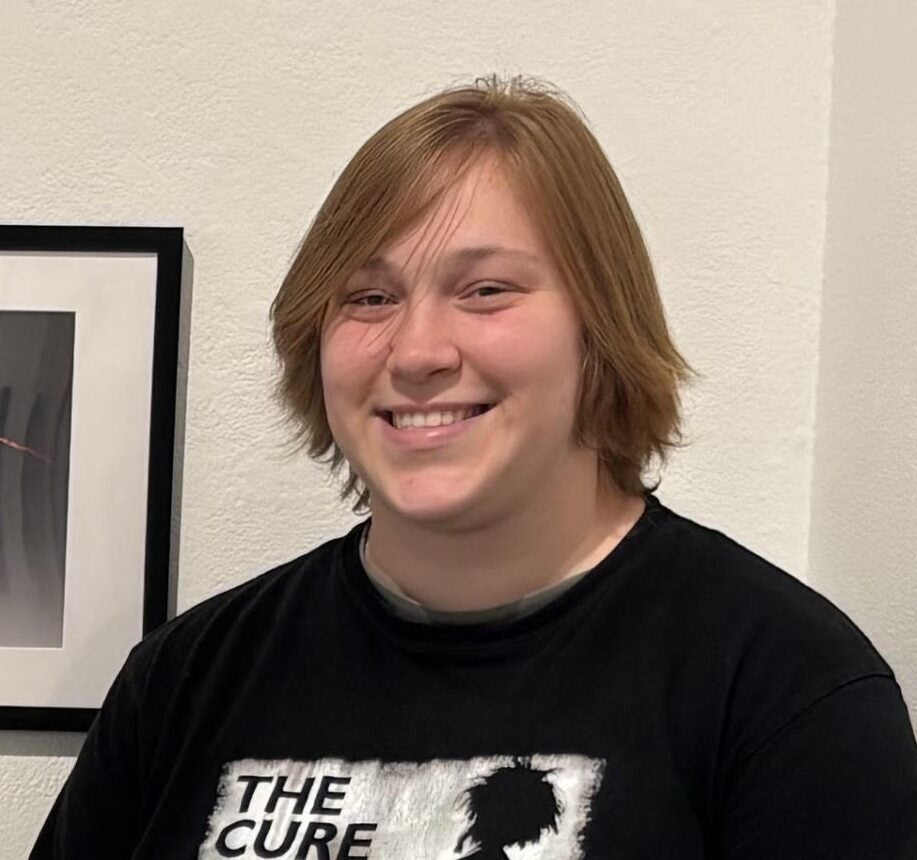
Mac Oliveau
Science & Technology Writer | AI and Robotics Reporter
Mac Oliveau is a Los Angeles–based science and technology journalist for The Brighter Side of News, an online publication focused on uplifting, transformative stories from around the globe. Passionate about spotlighting groundbreaking discoveries and innovations, Mac covers a broad spectrum of topics—from medical breakthroughs and artificial intelligence to green tech and archeology. With a talent for making complex science clear and compelling, they connect readers to the advancements shaping a brighter, more hopeful future.